import pandas as pd
import numpy as np
import matplotlib.pyplot as plt
import seaborn as sns
from matplotlib_inline.backend_inline import set_matplotlib_formats
from IPython.display import display, IFrame
# Pandas Tutor setup
%reload_ext pandas_tutor
%set_pandas_tutor_options {"maxDisplayCols": 8, "nohover": True, "projectorMode": True}
set_matplotlib_formats("svg")
sns.set_context("poster")
sns.set_style("whitegrid")
plt.rcParams["figure.figsize"] = (10, 5)
pd.set_option("display.max_rows", 8)
pd.set_option("display.max_columns", 8)
pd.set_option("display.precision", 2)
def show_paradox_slides():
src = 'https://docs.google.com/presentation/d/e/2PACX-1vSbFSaxaYZ0NcgrgqZLvjhkjX-5MQzAITWAsEFZHnix3j1c0qN8Vd1rogTAQP7F7Nf5r-JWExnGey7h/embed?start=false'
width = 960
height = 569
display(IFrame(src, width, height))
📣 Announcements 📣¶
- Good job turning in Lab 1!
- Lab 2 out, due Monday.
- Project 1 checkpoint due tomorrow.
- Project 1 due next Wed.
Data granularity¶
Example: Baby Names¶
What is a single observation in the baby names data?
baby = pd.read_csv('data/baby.csv')
baby
Name | Sex | Count | Year | |
---|---|---|---|---|
0 | Liam | M | 20456 | 2022 |
1 | Noah | M | 18621 | 2022 |
2 | Olivia | F | 16573 | 2022 |
3 | Oliver | M | 15076 | 2022 |
... | ... | ... | ... | ... |
2085154 | Worthy | M | 5 | 1880 |
2085155 | Wright | M | 5 | 1880 |
2085156 | York | M | 5 | 1880 |
2085157 | Zachariah | M | 5 | 1880 |
2085158 rows × 4 columns
Example: CO2 readings¶
What is a single observation in this dataset of CO2 readings?
# Don't about this code, we'll cover it when we talk about data cleaning
co2 = pd.read_csv('data/co2_mm_mlo.txt',
header=None, skiprows=72, sep='\s+',
names=['Yr', 'Mo', 'DecDate', 'Avg', 'co2', 'Trend', 'days'],
usecols=['Yr', 'Mo', 'DecDate', 'co2'])
co2
Yr | Mo | DecDate | co2 | |
---|---|---|---|---|
0 | 1958 | 3 | 1958.21 | 315.71 |
1 | 1958 | 4 | 1958.29 | 317.45 |
2 | 1958 | 5 | 1958.38 | 317.50 |
3 | 1958 | 6 | 1958.46 | 317.10 |
... | ... | ... | ... | ... |
734 | 2019 | 5 | 2019.38 | 414.66 |
735 | 2019 | 6 | 2019.46 | 413.92 |
736 | 2019 | 7 | 2019.54 | 411.77 |
737 | 2019 | 8 | 2019.62 | 409.95 |
738 rows × 4 columns
Example: CO2 readings by month¶
# Don't worry about understanding this code for now
sns.lineplot(data=co2, x='DecDate', y='co2');
Collecting data¶
- If you can control how your dataset is created, you should opt for finer granularity, i.e. for more detail.
- You can easily remove detail, but it's difficult to add detail if it is not already present in the dataset.
- Tradeoff: obtaining fine-grained data can take more time/money.
Manipulating granularity¶
- We'll now explore how to change the level of granularity present in our dataset.
- While it may seem like we are "losing information," removing detail can help us understand bigger-picture trends in our data.
Example: Penguins¶
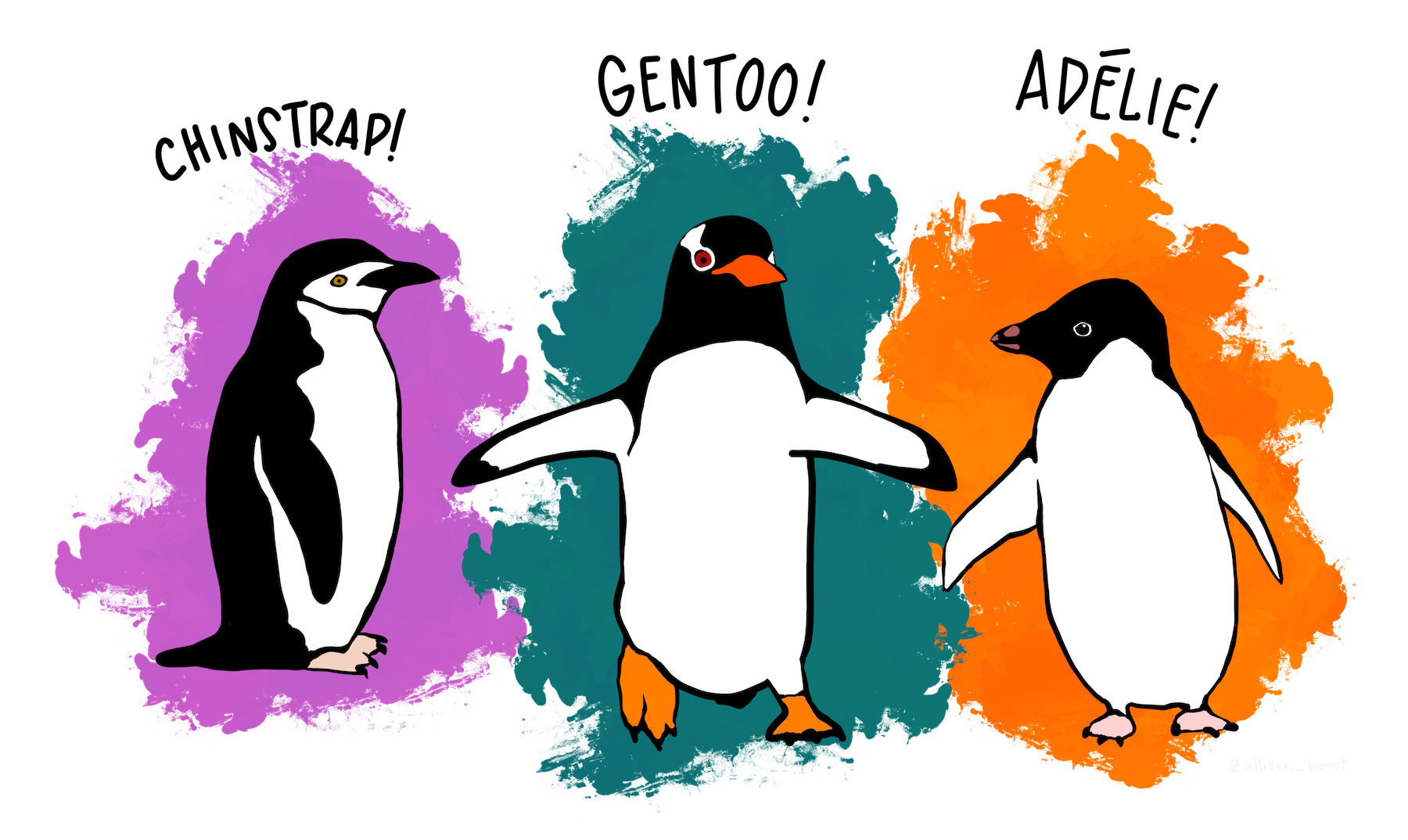
The dataset we'll work with for the rest of the lecture involves various measurements taken of three species of penguins in Antarctica.
import seaborn as sns
penguins = sns.load_dataset('penguins').dropna()
penguins
species | island | bill_length_mm | bill_depth_mm | flipper_length_mm | body_mass_g | sex | |
---|---|---|---|---|---|---|---|
0 | Adelie | Torgersen | 39.1 | 18.7 | 181.0 | 3750.0 | Male |
1 | Adelie | Torgersen | 39.5 | 17.4 | 186.0 | 3800.0 | Female |
2 | Adelie | Torgersen | 40.3 | 18.0 | 195.0 | 3250.0 | Female |
4 | Adelie | Torgersen | 36.7 | 19.3 | 193.0 | 3450.0 | Female |
... | ... | ... | ... | ... | ... | ... | ... |
340 | Gentoo | Biscoe | 46.8 | 14.3 | 215.0 | 4850.0 | Female |
341 | Gentoo | Biscoe | 50.4 | 15.7 | 222.0 | 5750.0 | Male |
342 | Gentoo | Biscoe | 45.2 | 14.8 | 212.0 | 5200.0 | Female |
343 | Gentoo | Biscoe | 49.9 | 16.1 | 213.0 | 5400.0 | Male |
333 rows × 7 columns
# What is the distribution of different 'species' in this dataset?
penguins['species'].value_counts()
Adelie 146 Gentoo 119 Chinstrap 68 Name: species, dtype: int64
# Overall, what is the distribution of different islands?
penguins['island'].value_counts()
Biscoe 163 Dream 123 Torgersen 47 Name: island, dtype: int64
Video: Palmer Penguins¶
IFrame('https://www.youtube-nocookie.com/embed/CCrNAHXUstU?si=-DntSyUNp5Kwitjm&start=11',
width=560, height=315)
Aggregating: Basics¶
We know how to find the mean body mass for all the penguins:
penguins['body_mass_g'].mean()
4207.057057057057
💡 Pro-Tip: Using f-strings¶
Python f-strings give an easy way to print variables nicely:
mean_body_mass = penguins['body_mass_g'].mean()
print(f'Mean penguin mass: {mean_body_mass:.2f} grams')
Mean penguin mass: 4207.06 grams
Aggregating: Basics¶
But what about the mean for each type of penguin?
penguins['body_mass_g'].mean()
4207.057057057057
Naive approach: looping through unique values¶
species_map = pd.Series([], dtype=float)
for species in penguins['species'].unique():
species_only = penguins.loc[penguins['species'] == species]
species_map.loc[species] = species_only['body_mass_g'].mean()
species_map
Adelie 3706.16 Chinstrap 3733.09 Gentoo 5092.44 dtype: float64
For each unique
'species'
, we make a pass through the entire dataset.- The asymptotic runtime of this procedure is $\Theta(ns)$, where $n$ is the number of rows and $s$ is the number of unique species.
While there are other loop-based solutions that only involve a single pass over the DataFrame, we'd like to avoid Python loops entirely, as they're slow.
Grouping¶
# Before:
penguins['body_mass_g'].mean()
# After:
penguins.groupby('species')['body_mass_g'].mean()
species Adelie 3706.16 Chinstrap 3733.09 Gentoo 5092.44 Name: body_mass_g, dtype: float64
Somehow, the groupby
method computes what we're looking for in just one line. How?
%%pt
penguins.groupby('species')['body_mass_g'].mean()
"Split-apply-combine" paradigm¶
The groupby
method involves three steps: split, apply, and combine. This is the same terminology that the pandas
documentation uses.
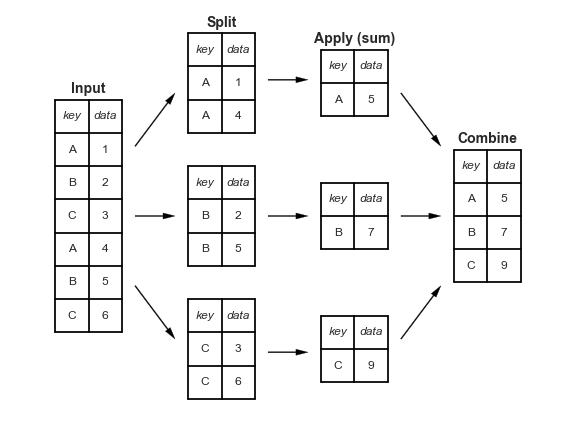
Split breaks up and "groups" the rows of a DataFrame according to the specified key. There is one "group" for every unique value of the key.
Apply uses a function (e.g. aggregation, transformation, filtration) within the individual groups.
Combine stitches the results of these operations into an output DataFrame.
The split-apply-combine pattern can be parallelized to work on multiple computers or threads, by sending computations for each group to different processors.
More examples¶
Before we dive into the internals, let's look at a few more examples.
penguins.head()
species | island | bill_length_mm | bill_depth_mm | flipper_length_mm | body_mass_g | sex | |
---|---|---|---|---|---|---|---|
0 | Adelie | Torgersen | 39.1 | 18.7 | 181.0 | 3750.0 | Male |
1 | Adelie | Torgersen | 39.5 | 17.4 | 186.0 | 3800.0 | Female |
2 | Adelie | Torgersen | 40.3 | 18.0 | 195.0 | 3250.0 | Female |
4 | Adelie | Torgersen | 36.7 | 19.3 | 193.0 | 3450.0 | Female |
5 | Adelie | Torgersen | 39.3 | 20.6 | 190.0 | 3650.0 | Male |
penguins.shape
(333, 7)
Which 'species'
has the highest median 'bill_length_mm'
?
(penguins
.groupby('species')
.median()
['bill_length_mm']
.idxmax()
)
'Chinstrap'
What proportion of penguins of each 'species'
live on 'Dream'
island?
(penguins
.assign(on_dream = penguins['island'] == 'Dream')
.groupby('species')
.mean()['on_dream']
)
species Adelie 0.38 Chinstrap 1.00 Gentoo 0.00 Name: on_dream, dtype: float64
DataFrameGroupBy
objects and aggregation¶
DataFrameGroupBy
objects¶
We've just evaluated a few expressions of the following form.
penguins.groupby('species').mean()
bill_length_mm | bill_depth_mm | flipper_length_mm | body_mass_g | |
---|---|---|---|---|
species | ||||
Adelie | 38.82 | 18.35 | 190.10 | 3706.16 |
Chinstrap | 48.83 | 18.42 | 195.82 | 3733.09 |
Gentoo | 47.57 | 15.00 | 217.24 | 5092.44 |
There are two method calls in the expression above: .groupby('species')
and .mean()
. What happens if we remove the latter?
penguins.groupby('species')
<pandas.core.groupby.generic.DataFrameGroupBy object at 0x7fb8c011e070>
Peeking under the hood¶
If df
is a DataFrame, then df.groupby(key)
returns a DataFrameGroupBy
object.
This object represents the "split" in "split-apply-combine".
# Simplified table for demostration:
penguins_small = penguins.iloc[[0, 1, 150, 151, 251, 300, 301], [0, 5, 6]]
# Creates one group for each unique value in the species column.
penguin_groups = penguins_small.groupby('species')
penguin_groups
<pandas.core.groupby.generic.DataFrameGroupBy object at 0x7fb8c00ed640>
%%pt
penguin_groups
DataFrameGroupBy
objects have a groups
attribute, which is a dictionary in which the keys are group names and the values are lists of row labels.
penguin_groups.groups
{'Adelie': [0, 1], 'Chinstrap': [156, 157], 'Gentoo': [258, 308, 309]}
DataFrameGroupBy
objects also have a get_group(key)
method, which returns a DataFrame with only the values for the given key.
penguin_groups.get_group('Chinstrap')
species | body_mass_g | sex | |
---|---|---|---|
156 | Chinstrap | 3725.0 | Male |
157 | Chinstrap | 3950.0 | Female |
# Same as the above!
penguins_small.query('species == "Chinstrap"')
species | body_mass_g | sex | |
---|---|---|---|
156 | Chinstrap | 3725.0 | Male |
157 | Chinstrap | 3950.0 | Female |
We usually don't use these attributes and methods, but they're useful in understanding how groupby
works under the hood.
Aggregation¶
Once we create a
DataFrameGroupBy
object, we need to apply some function to each group, and combine the results.The most common operation we apply to each group is an aggregation.
- Aggregation refers to the process of reducing many values to one.
To perform an aggregation, use an aggregation method on the
DataFrameGroupBy
object, e.g..mean()
,.max()
, or.median()
.
Let's look at some examples.
penguins_small
species | body_mass_g | sex | |
---|---|---|---|
0 | Adelie | 3750.0 | Male |
1 | Adelie | 3800.0 | Female |
156 | Chinstrap | 3725.0 | Male |
157 | Chinstrap | 3950.0 | Female |
258 | Gentoo | 4350.0 | Female |
308 | Gentoo | 4875.0 | Female |
309 | Gentoo | 5550.0 | Male |
penguins_small.groupby('species').mean()
body_mass_g | |
---|---|
species | |
Adelie | 3775.0 |
Chinstrap | 3837.5 |
Gentoo | 4925.0 |
penguins_small.groupby('species').sum()
body_mass_g | |
---|---|
species | |
Adelie | 7550.0 |
Chinstrap | 7675.0 |
Gentoo | 14775.0 |
penguins_small.groupby('species').last()
body_mass_g | sex | |
---|---|---|
species | ||
Adelie | 3800.0 | Female |
Chinstrap | 3950.0 | Female |
Gentoo | 5550.0 | Male |
penguins_small.groupby('species').max()
body_mass_g | sex | |
---|---|---|
species | ||
Adelie | 3800.0 | Male |
Chinstrap | 3950.0 | Male |
Gentoo | 5550.0 | Male |
Column independence¶
Within each group, the aggregation method is applied to each column independently.
penguins_small.groupby('species').max()
body_mass_g | sex | |
---|---|---|
species | ||
Adelie | 3800.0 | Male |
Chinstrap | 3950.0 | Male |
Gentoo | 5550.0 | Male |
It is not telling us that there is a male 'Adelie'
penguin with a 'body_mass_g'
of 3800.0
!
# This penguin is Female!
penguins_small.loc[(penguins['species'] == 'Adelie') & (penguins['body_mass_g'] == 3800.0)]
species | body_mass_g | sex | |
---|---|---|---|
1 | Adelie | 3800.0 | Female |
Discussion Question¶
Find the species and weights of the heaviest Male
and Female
penguins.
# Fill in this cell
Column selection and performance implications¶
By default, the aggregator will be applied to all columns that it can be applied to.
max
andmin
are defined on strings, whilemedian
andmean
are not.
If we only care about one column, we can select that column before aggregating to save time.
DataFrameGroupBy
objects support[]
notation, just likeDataFrame
s.
# Back to the big penguins dataset
penguins.groupby('species').mean()
bill_length_mm | bill_depth_mm | flipper_length_mm | body_mass_g | |
---|---|---|---|---|
species | ||||
Adelie | 38.82 | 18.35 | 190.10 | 3706.16 |
Chinstrap | 48.83 | 18.42 | 195.82 | 3733.09 |
Gentoo | 47.57 | 15.00 | 217.24 | 5092.44 |
# Works, but involves wasted effort since the other columns had to be aggregated for no reason.
penguins.groupby('species').mean()['bill_length_mm']
species Adelie 38.82 Chinstrap 48.83 Gentoo 47.57 Name: bill_length_mm, dtype: float64
# This is a SeriesGroupBy object!
penguins.groupby('species')['bill_length_mm']
<pandas.core.groupby.generic.SeriesGroupBy object at 0x7fb8d46c14f0>
# Saves time!
penguins.groupby('species')['bill_length_mm'].mean()
species Adelie 38.82 Chinstrap 48.83 Gentoo 47.57 Name: bill_length_mm, dtype: float64
To demonstrate that the former is slower than the latter, we can use %%timeit
. For reference, we'll also include our earlier for
-loop-based solution.
%%timeit
penguins.groupby('species').mean()['bill_length_mm']
359 µs ± 1.94 µs per loop (mean ± std. dev. of 7 runs, 1,000 loops each)
%%timeit
penguins.groupby('species')['bill_length_mm'].mean()
137 µs ± 659 ns per loop (mean ± std. dev. of 7 runs, 10,000 loops each)
%%timeit
species_map = pd.Series([], dtype=float)
for species in penguins['species'].unique():
species_only = penguins.loc[penguins['species'] == species]
species_map.loc[species] = species_only['body_mass_g'].mean()
species_map
981 µs ± 1.88 µs per loop (mean ± std. dev. of 7 runs, 1,000 loops each)
Takeaways¶
It's important to understand what each piece of your code evaluates to – in the first two timed examples, the code is almost identical, but the performance is quite different.
# Slower penguins.groupby('species').mean()['bill_length_mm'] # Faster penguins.groupby('species')['bill_length_mm'].mean()
The
groupby
method is much quicker thanfor
-looping over the DataFrame in Python. It can often produce results using just a single, fast pass over the data, updating the sum, mean, count, min, or other aggregate for each group along the way.
Beyond default aggregation methods¶
- There are many built-in aggregation methods.
- What if you want to apply different aggregation methods to different columns?
- What if the aggregation method you want to use doesn't already exist in
pandas
?
The aggregate
method¶
- The
DataFrameGroupBy
object has a generalaggregate
method, which aggregates using one or more operations.- Remember, aggregation refers to the process of reducing many values to one.
- There are many ways of using
aggregate
; refer to the documentation for a comprehensive list. - Example arguments:
- A single function.
- A list of functions.
- A dictionary mapping column names to functions.
- Per the documentation,
agg
is an alias foraggregate
.
Example¶
How many penguins are there of each 'species'
, and what is the mean 'body_mass_g'
of each species?
(penguins
.groupby('species')
['body_mass_g']
.aggregate(['count', 'mean'])
)
count | mean | |
---|---|---|
species | ||
Adelie | 146 | 3706.16 |
Chinstrap | 68 | 3733.09 |
Gentoo | 119 | 5092.44 |
Note what happens when we don't select a column before aggregating.
(penguins
.groupby('species')
.aggregate(['count', 'mean'])
)
bill_length_mm | bill_depth_mm | flipper_length_mm | body_mass_g | |||||
---|---|---|---|---|---|---|---|---|
count | mean | count | mean | count | mean | count | mean | |
species | ||||||||
Adelie | 146 | 38.82 | 146 | 18.35 | 146 | 190.10 | 146 | 3706.16 |
Chinstrap | 68 | 48.83 | 68 | 18.42 | 68 | 195.82 | 68 | 3733.09 |
Gentoo | 119 | 47.57 | 119 | 15.00 | 119 | 217.24 | 119 | 5092.44 |
Example¶
What is the maximum 'bill_length_mm'
of each species, and which 'island'
s is each 'species'
found on?
(penguins
.groupby('species')
.aggregate({'bill_length_mm': 'max', 'island': 'nunique'})
)
bill_length_mm | island | |
---|---|---|
species | ||
Adelie | 46.0 | 3 |
Chinstrap | 58.0 | 1 |
Gentoo | 59.6 | 1 |
Example¶
What is the interquartile range of the 'body_mass_g'
of each 'species'
?
# The function for .agg takes in a pd.Series as its argument and returns a scalar
def iqr(series):
return np.percentile(series, 75) - np.percentile(series, 25)
(penguins
.groupby('species')
['body_mass_g']
.agg(iqr)
)
species Adelie 637.5 Chinstrap 462.5 Gentoo 800.0 Name: body_mass_g, dtype: float64
Other DataFrameGroupBy
methods¶
Split-apply-combine, revisited¶
When we introduced the split-apply-combine pattern, the "apply" step involved aggregation – our final DataFrame had one row for each group.
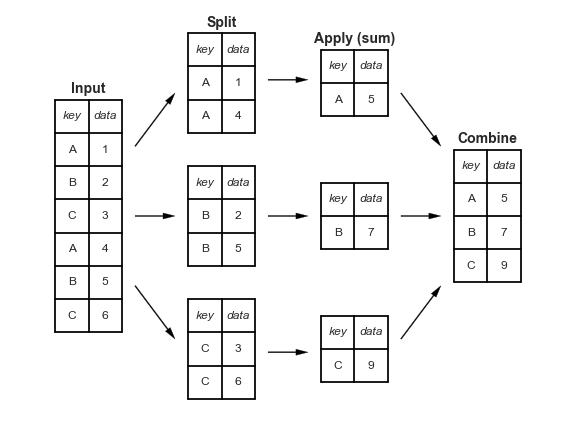
Instead of aggregating during the apply step, we could instead perform a:
Transformation, in which we perform operations to every value within each group.
Filtration, in which we keep only the groups that satisfy some condition.
Transformations¶
- Suppose we want to convert the
'body_mass_g'
column to to z-scores (i.e. standard units):
def z_score(x):
return (x - x.mean()) / x.std(ddof=0)
z_score(penguins['body_mass_g'])
0 -0.57 1 -0.51 2 -1.19 4 -0.94 ... 340 0.80 341 1.92 342 1.23 343 1.48 Name: body_mass_g, Length: 333, dtype: float64
Transformations within groups¶
Now, what if we wanted the z-score within each group?
To do so, we can use the
transform
method on aDataFrameGroupBy
object. Thetransform
method takes in a function, which itself takes in a Series and returns a new Series.A transformation produces a DataFrame or Series of the same size – it is not an aggregation!
z_mass = (penguins
.groupby('species')
['body_mass_g']
.transform(z_score))
z_mass
0 0.10 1 0.21 2 -1.00 4 -0.56 ... 340 -0.49 341 1.32 342 0.22 343 0.62 Name: body_mass_g, Length: 333, dtype: float64
penguins.assign(z_mass=z_mass)
species | island | bill_length_mm | bill_depth_mm | flipper_length_mm | body_mass_g | sex | z_mass | |
---|---|---|---|---|---|---|---|---|
0 | Adelie | Torgersen | 39.1 | 18.7 | 181.0 | 3750.0 | Male | 0.10 |
1 | Adelie | Torgersen | 39.5 | 17.4 | 186.0 | 3800.0 | Female | 0.21 |
2 | Adelie | Torgersen | 40.3 | 18.0 | 195.0 | 3250.0 | Female | -1.00 |
4 | Adelie | Torgersen | 36.7 | 19.3 | 193.0 | 3450.0 | Female | -0.56 |
... | ... | ... | ... | ... | ... | ... | ... | ... |
340 | Gentoo | Biscoe | 46.8 | 14.3 | 215.0 | 4850.0 | Female | -0.49 |
341 | Gentoo | Biscoe | 50.4 | 15.7 | 222.0 | 5750.0 | Male | 1.32 |
342 | Gentoo | Biscoe | 45.2 | 14.8 | 212.0 | 5200.0 | Female | 0.22 |
343 | Gentoo | Biscoe | 49.9 | 16.1 | 213.0 | 5400.0 | Male | 0.62 |
333 rows × 8 columns
Note that below, penguin 340 has a larger 'body_mass_g'
than penguin 0, but a lower 'z_mass'
.
- Penguin 0 has an above average
'body_mass_g'
among'Adelie'
penguins. - Penguin 340 has a below average
'body_mass_g'
among'Gentoo'
penguins. Remember from earlier that the average'body_mass_g'
of'Gentoo'
penguins is much higher than for other species.
Filtering Groups¶
To keep only the groups that satisfy a particular condition, use the
filter
method on aDataFrameGroupBy
object.The
filter
method takes in a function, which itself takes in a DataFrame/Series and return a single Boolean. The result is a new DataFrame/Series with only the groups for which the filter function returnedTrue
.
For example, suppose we want only the 'species'
whose average 'bill_length_mm'
is above 39.
(penguins
.groupby('species')
.filter(lambda df: df['bill_length_mm'].mean() > 39)
)
species | island | bill_length_mm | bill_depth_mm | flipper_length_mm | body_mass_g | sex | |
---|---|---|---|---|---|---|---|
152 | Chinstrap | Dream | 46.5 | 17.9 | 192.0 | 3500.0 | Female |
153 | Chinstrap | Dream | 50.0 | 19.5 | 196.0 | 3900.0 | Male |
154 | Chinstrap | Dream | 51.3 | 19.2 | 193.0 | 3650.0 | Male |
155 | Chinstrap | Dream | 45.4 | 18.7 | 188.0 | 3525.0 | Female |
... | ... | ... | ... | ... | ... | ... | ... |
340 | Gentoo | Biscoe | 46.8 | 14.3 | 215.0 | 4850.0 | Female |
341 | Gentoo | Biscoe | 50.4 | 15.7 | 222.0 | 5750.0 | Male |
342 | Gentoo | Biscoe | 45.2 | 14.8 | 212.0 | 5200.0 | Female |
343 | Gentoo | Biscoe | 49.9 | 16.1 | 213.0 | 5400.0 | Male |
187 rows × 7 columns
No more 'Adelie'
s!
Or, as another example, suppose we only want 'species'
with at least 100 penguins:
penguins.groupby('species').filter(lambda df: df.shape[0] > 100)
species | island | bill_length_mm | bill_depth_mm | flipper_length_mm | body_mass_g | sex | |
---|---|---|---|---|---|---|---|
0 | Adelie | Torgersen | 39.1 | 18.7 | 181.0 | 3750.0 | Male |
1 | Adelie | Torgersen | 39.5 | 17.4 | 186.0 | 3800.0 | Female |
2 | Adelie | Torgersen | 40.3 | 18.0 | 195.0 | 3250.0 | Female |
4 | Adelie | Torgersen | 36.7 | 19.3 | 193.0 | 3450.0 | Female |
... | ... | ... | ... | ... | ... | ... | ... |
340 | Gentoo | Biscoe | 46.8 | 14.3 | 215.0 | 4850.0 | Female |
341 | Gentoo | Biscoe | 50.4 | 15.7 | 222.0 | 5750.0 | Male |
342 | Gentoo | Biscoe | 45.2 | 14.8 | 212.0 | 5200.0 | Female |
343 | Gentoo | Biscoe | 49.9 | 16.1 | 213.0 | 5400.0 | Male |
265 rows × 7 columns
No more 'Chinstrap'
s!
Grouping with multiple columns¶
When we group with multiple columns, one group is created for every unique combination of elements in the specified columns.
species_and_island = penguins.groupby(['species', 'island']).mean()
species_and_island
bill_length_mm | bill_depth_mm | flipper_length_mm | body_mass_g | ||
---|---|---|---|---|---|
species | island | ||||
Adelie | Biscoe | 38.98 | 18.37 | 188.80 | 3709.66 |
Dream | 38.52 | 18.24 | 189.93 | 3701.36 | |
Torgersen | 39.04 | 18.45 | 191.53 | 3708.51 | |
Chinstrap | Dream | 48.83 | 18.42 | 195.82 | 3733.09 |
Gentoo | Biscoe | 47.57 | 15.00 | 217.24 | 5092.44 |
Grouping and indexes¶
- The
groupby
method creates an index based on the specified columns. - When grouping by multiple columns, the resulting DataFrame has a
MultiIndex
. - Advice: When working with a
MultiIndex
, usereset_index
or setas_index=False
ingroupby
.
species_and_island
bill_length_mm | bill_depth_mm | flipper_length_mm | body_mass_g | ||
---|---|---|---|---|---|
species | island | ||||
Adelie | Biscoe | 38.98 | 18.37 | 188.80 | 3709.66 |
Dream | 38.52 | 18.24 | 189.93 | 3701.36 | |
Torgersen | 39.04 | 18.45 | 191.53 | 3708.51 | |
Chinstrap | Dream | 48.83 | 18.42 | 195.82 | 3733.09 |
Gentoo | Biscoe | 47.57 | 15.00 | 217.24 | 5092.44 |
species_and_island['body_mass_g']
species island Adelie Biscoe 3709.66 Dream 3701.36 Torgersen 3708.51 Chinstrap Dream 3733.09 Gentoo Biscoe 5092.44 Name: body_mass_g, dtype: float64
species_and_island.loc['Adelie']
bill_length_mm | bill_depth_mm | flipper_length_mm | body_mass_g | |
---|---|---|---|---|
island | ||||
Biscoe | 38.98 | 18.37 | 188.80 | 3709.66 |
Dream | 38.52 | 18.24 | 189.93 | 3701.36 |
Torgersen | 39.04 | 18.45 | 191.53 | 3708.51 |
species_and_island.loc[('Adelie', 'Torgersen')]
bill_length_mm 39.04 bill_depth_mm 18.45 flipper_length_mm 191.53 body_mass_g 3708.51 Name: (Adelie, Torgersen), dtype: float64
species_and_island.reset_index()
species | island | bill_length_mm | bill_depth_mm | flipper_length_mm | body_mass_g | |
---|---|---|---|---|---|---|
0 | Adelie | Biscoe | 38.98 | 18.37 | 188.80 | 3709.66 |
1 | Adelie | Dream | 38.52 | 18.24 | 189.93 | 3701.36 |
2 | Adelie | Torgersen | 39.04 | 18.45 | 191.53 | 3708.51 |
3 | Chinstrap | Dream | 48.83 | 18.42 | 195.82 | 3733.09 |
4 | Gentoo | Biscoe | 47.57 | 15.00 | 217.24 | 5092.44 |
penguins.groupby(['species', 'island'], as_index=False).mean()
species | island | bill_length_mm | bill_depth_mm | flipper_length_mm | body_mass_g | |
---|---|---|---|---|---|---|
0 | Adelie | Biscoe | 38.98 | 18.37 | 188.80 | 3709.66 |
1 | Adelie | Dream | 38.52 | 18.24 | 189.93 | 3701.36 |
2 | Adelie | Torgersen | 39.04 | 18.45 | 191.53 | 3708.51 |
3 | Chinstrap | Dream | 48.83 | 18.42 | 195.82 | 3733.09 |
4 | Gentoo | Biscoe | 47.57 | 15.00 | 217.24 | 5092.44 |
Discussion: Checking your knowledge¶
Find the most popular male and female baby name for each year in the dataset. Exclude years where there were fewer than 1 million births recorded.
baby = pd.read_csv('data/baby.csv')
baby
Name | Sex | Count | Year | |
---|---|---|---|---|
0 | Liam | M | 20456 | 2022 |
1 | Noah | M | 18621 | 2022 |
2 | Olivia | F | 16573 | 2022 |
3 | Oliver | M | 15076 | 2022 |
... | ... | ... | ... | ... |
2085154 | Worthy | M | 5 | 1880 |
2085155 | Wright | M | 5 | 1880 |
2085156 | York | M | 5 | 1880 |
2085157 | Zachariah | M | 5 | 1880 |
2085158 rows × 4 columns
# Fill me in
Pivot Tables: An extension of grouping¶
Pivot tables are a compact way to display tables for humans to read:
Sex | F | M |
---|---|---|
Year | ||
2018 | 1698373 | 1813377 |
2019 | 1675139 | 1790682 |
2020 | 1612393 | 1721588 |
2021 | 1635800 | 1743913 |
2022 | 1628730 | 1733166 |
- Notice that each value in the table is a sum over the counts, split by year and sex.
- You can think of pivot tables as grouping using two columns, then "pivoting" one of the group keys into columns
pivot_table
¶
The pivot_table
DataFrame method aggregates a DataFrame using two columns. To use it:
df.pivot_table(index=index_col,
columns=columns_col,
values=values_col,
aggfunc=func)
The resulting DataFrame will have:
- One row for every unique value in
index_col
. - One column for every unique value in
columns_col
. - Values determined by applying
func
on values invalues_col
.
last_5_years = baby.query('Year >= 2018')
last_5_years.pivot_table(
index='Year',
columns='Sex',
values='Count',
aggfunc='sum',
)
Sex | F | M |
---|---|---|
Year | ||
2018 | 1698373 | 1813377 |
2019 | 1675139 | 1790682 |
2020 | 1612393 | 1721588 |
2021 | 1635800 | 1743913 |
2022 | 1628730 | 1733166 |
# Look at the similarity to the snippet above
(last_5_years
.groupby(['Year', 'Sex'])
['Count']
.sum()
.unstack('Sex')
)
Sex | F | M |
---|---|---|
Year | ||
2018 | 1698373 | 1813377 |
2019 | 1675139 | 1790682 |
2020 | 1612393 | 1721588 |
2021 | 1635800 | 1743913 |
2022 | 1628730 | 1733166 |
Example:¶
Find the number of penguins per island and species.
penguins.pivot_table(
index='species',
columns='island',
values='bill_length_mm', # Choice of column here doesn't actually matter
aggfunc='count',
)
island | Biscoe | Dream | Torgersen |
---|---|---|---|
species | |||
Adelie | 44.0 | 55.0 | 47.0 |
Chinstrap | NaN | 68.0 | NaN |
Gentoo | 119.0 | NaN | NaN |
Note that there is a NaN
at the intersection of 'Biscoe'
and 'Chinstrap'
, because there were no Chinstrap penguins on Biscoe Island.
We can either use the fillna
method afterwards or the fill_value
argument to fill in NaN
s.
penguins.pivot_table(
index='species',
columns='island',
values='bill_length_mm',
aggfunc='count',
fill_value=0,
)
island | Biscoe | Dream | Torgersen |
---|---|---|---|
species | |||
Adelie | 44 | 55 | 47 |
Chinstrap | 0 | 68 | 0 |
Gentoo | 119 | 0 | 0 |
Granularity, revisited¶
Take another look at the pivot table from the previous cell. Each row of the original penguins
represented a single penguin, and each column represented features of the penguins.
What is the granularity of this table?
penguins.pivot_table(
index='species',
columns='island',
values='bill_length_mm',
aggfunc='count',
fill_value=0,
)
island | Biscoe | Dream | Torgersen |
---|---|---|---|
species | |||
Adelie | 44 | 55 | 47 |
Chinstrap | 0 | 68 | 0 |
Gentoo | 119 | 0 | 0 |
Reshaping¶
pivot_table
reshapes DataFrames from "long" to "wide".- Other DataFrame reshaping methods:
melt
: Un-pivots a DataFrame. Very useful in data cleaning.pivot
: Likepivot_table
, but doesn't do aggregation.stack
: Pivots multi-level columns to multi-indices.unstack
: Pivots multi-indices to columns.- Google and the documentation are your friends!
Distributions¶
Joint distribution¶
When using aggfunc='count'
, a pivot table describes the joint distribution of two categorical variables. This is also called a contingency table.
counts = penguins.pivot_table(
index='species',
columns='sex',
values='body_mass_g',
aggfunc='count',
fill_value=0
)
counts
sex | Female | Male |
---|---|---|
species | ||
Adelie | 73 | 73 |
Chinstrap | 34 | 34 |
Gentoo | 58 | 61 |
We can normalize the DataFrame by dividing by the total number of penguins. The resulting numbers can be interpreted as probabilities that a randomly selected penguin from the dataset belongs to a given combination of species and sex.
joint = counts / counts.sum().sum()
joint
sex | Female | Male |
---|---|---|
species | ||
Adelie | 0.22 | 0.22 |
Chinstrap | 0.10 | 0.10 |
Gentoo | 0.17 | 0.18 |
Marginal probabilities¶
If we sum over one of the axes, we can compute marginal probabilities, i.e. unconditional probabilities.
joint
sex | Female | Male |
---|---|---|
species | ||
Adelie | 0.22 | 0.22 |
Chinstrap | 0.10 | 0.10 |
Gentoo | 0.17 | 0.18 |
# Recall, joint.sum(axis=0) sums across the rows, which computes the sum of the **columns**.
joint.sum(axis=0)
sex Female 0.5 Male 0.5 dtype: float64
joint.sum(axis=1)
species Adelie 0.44 Chinstrap 0.20 Gentoo 0.36 dtype: float64
For instance, the second Series tells us that a randomly selected penguin has a 0.36 chance of being of species 'Gentoo'
.
Conditional probabilities¶
Using counts
, how might we compute conditional probabilities like $$P(\text{species } = \text{"Adelie"} \mid \text{sex } = \text{"Female"})?$$
counts
sex | Female | Male |
---|---|---|
species | ||
Adelie | 73 | 73 |
Chinstrap | 34 | 34 |
Gentoo | 58 | 61 |
Answer: To find conditional probabilities of species given sex, divide by column sums. To find conditional probabilities of sex given species, divide by row sums.
Conditional probabilities¶
To find conditional probabilities of species given sex, divide by column sums. To find conditional probabilities of sex given species, divide by row sums.
counts
sex | Female | Male |
---|---|---|
species | ||
Adelie | 73 | 73 |
Chinstrap | 34 | 34 |
Gentoo | 58 | 61 |
counts.sum(axis=0)
sex Female 165 Male 168 dtype: int64
The conditional distribution of species given sex is below. Note that in this new DataFrame, the 'Female'
and 'Male'
columns each sum to 1.
counts / counts.sum(axis=0)
sex | Female | Male |
---|---|---|
species | ||
Adelie | 0.44 | 0.43 |
Chinstrap | 0.21 | 0.20 |
Gentoo | 0.35 | 0.36 |
For instance, the above DataFrame tells us that the probability that a randomly selected penguin is of species 'Adelie'
given that they are of sex 'Female'
is 0.442424.
Exercise: Try and find the conditional distribution of sex given species.
Simpson's paradox¶
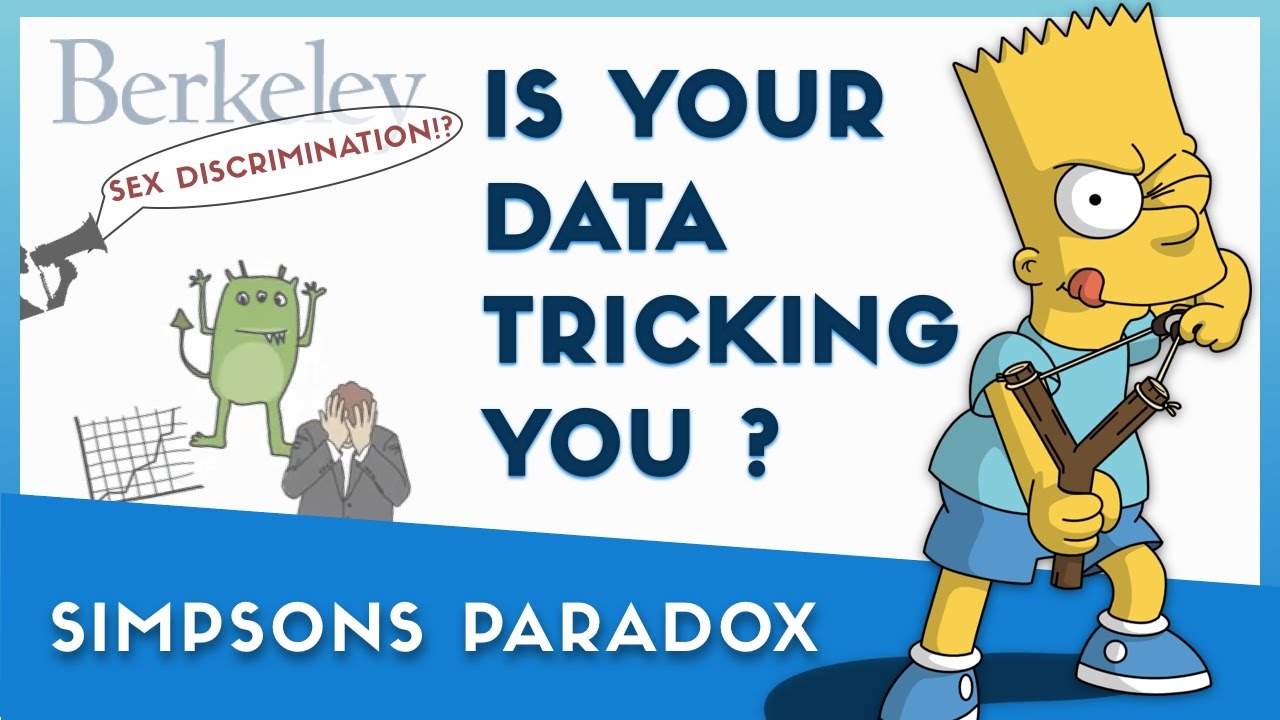
Example: Grades¶
Two students, Lisa and Bart, just finished freshman year. They both took a different number of classes in Fall, Winter, and Spring.
Each quarter, Lisa had a higher GPA than Bart.
But Bart has a higher overall GPA.
How is this possible? 🤔
Run this cell to create DataFrames that contain each students' grades.
lisa = pd.DataFrame([
[20, 46],
[18, 54],
[5, 20],
],
columns=['Units', 'Grade Points Earned'],
index=['Fall', 'Winter', 'Spring'],
)
bart = pd.DataFrame([
[5, 10],
[5, 13.5],
[22, 81.4],
],
columns=['Units', 'Grade Points Earned'],
index=['Fall', 'Winter', 'Spring'],
)
Quarter-specific vs. overall GPAs¶
Note: The number of "grade points" earned for a course is
$$\text{number of units} \cdot \text{grade (out of 4)}$$For instance, an A- in a 4 unit course earns $3.7 \cdot 4 = 14.8$ grade points.
lisa
Units | Grade Points Earned | |
---|---|---|
Fall | 20 | 46 |
Winter | 18 | 54 |
Spring | 5 | 20 |
bart
Units | Grade Points Earned | |
---|---|---|
Fall | 5 | 10.0 |
Winter | 5 | 13.5 |
Spring | 22 | 81.4 |
Lisa had a higher GPA in all three quarters:
quarterly_gpas = pd.DataFrame({
"Lisa's Quarter GPA": lisa['Grade Points Earned'] / lisa['Units'],
"Bart's Quarter GPA": bart['Grade Points Earned'] / bart['Units'],
})
quarterly_gpas
Lisa's Quarter GPA | Bart's Quarter GPA | |
---|---|---|
Fall | 2.3 | 2.0 |
Winter | 3.0 | 2.7 |
Spring | 4.0 | 3.7 |
But Lisa's overall GPA was less than Bart's overall GPA:
tot = lisa.sum()
tot['Grade Points Earned'] / tot['Units']
2.7906976744186047
tot = bart.sum()
tot['Grade Points Earned'] / tot['Units']
3.278125
What happened?¶
(quarterly_gpas
.assign(Lisa_units=lisa['Units'],
Bart_units=bart['Units'])
.iloc[:, [0, 2, 1, 3]]
)
Lisa's Quarter GPA | Lisa_units | Bart's Quarter GPA | Bart_units | |
---|---|---|---|---|
Fall | 2.3 | 20 | 2.0 | 5 |
Winter | 3.0 | 18 | 2.7 | 5 |
Spring | 4.0 | 5 | 3.7 | 22 |
When Lisa and Bart both performed poorly, Lisa took more units than Bart. This brought down 📉 Lisa's overall average.
When Lisa and Bart both performed well, Bart took more units than Annie. This brought up 📈 Bart's overall average.
Simpson's paradox¶
Simpson's paradox occurs when grouped data and ungrouped data show opposing trends.
- It is named after Edward H. Simpson, not Lisa or Bart Simpson.
It is purely arithmetic – it is a consequence of weighted averages.
It often happens because there is a hidden factor (i.e. a confounder) within the data that influences results.
Question: What is the "correct" way to summarize your data? What if you had to act on these results?
show_paradox_slides()
What happened?¶
The overall acceptance rate for women (30%) was lower than it was for men (45%).
However, most departments (A, B, D, F) had a higher acceptance rate for women.
Department A had a 62% acceptance rate for men and an 82% acceptance rate for women!
- 31% of men applied to Department A.
- 6% of women applied to Department A.
Department F had a 6% acceptance rate for men and a 7% acceptance rate for women!
- 14% of men applied to Department F.
- 19% of women applied to Department F.
Conclusion: Women tended to apply to departments with a lower acceptance rate; the data don't support the hypothesis that there was major gender discrimination against women.
Caution!¶
This doesn't mean that admissions are free from gender discrimination!
From Moss-Racusin et al., 2012, PNAS (cited 2600+ times):
In a randomized double-blind study (n = 127), science faculty from research-intensive universities rated the application materials of a student—who was randomly assigned either a male or female name—for a laboratory manager position. Faculty participants rated the male applicant as significantly more competent and hireable than the (identical) female applicant. These participants also selected a higher starting salary and offered more career mentoring to the male applicant. The gender of the faculty participants did not affect responses, such that female and male faculty were equally likely to exhibit bias against the female student.
But then...¶
From Williams and Ceci, 2015, PNAS:
Here we report five hiring experiments in which faculty evaluated hypothetical female and male applicants, using systematically varied profiles disguising identical scholarship, for assistant professorships in biology, engineering, economics, and psychology. Contrary to prevailing assumptions, men and women faculty members from all four fields preferred female applicants 2:1 over identically qualified males with matching lifestyles (single, married, divorced), with the exception of male economists, who showed no gender preference.
Do these conflict?¶
Not necessarily. One explanation, from William and Ceci:
Instead, past studies have used ratings of students’ hirability for a range of posts that do not include tenure-track jobs, such as managing laboratories or performing math assignments for a company. However, hiring tenure-track faculty differs from hiring lower-level staff: it entails selecting among highly accomplished candidates, all of whom have completed Ph.D.s and amassed publications and strong letters of support. Hiring bias may occur when applicants’ records are ambiguous, as was true in studies of hiring bias for lower-level staff posts, but such bias may not occur when records are clearly strong, as is the case with tenure-track hiring.
Do these conflict?¶
From Witteman, et al, 2019, in The Lancet:
Thus, evidence of scientists favouring women comes exclusively from hypothetical scenarios, whereas evidence of scientists favouring men comes from hypothetical scenarios and real behaviour. This might reflect academics' growing awareness of the social desirability of achieving gender balance, while real academic behaviour might not yet put such ideals into action.
Example: Restaurant reviews and phone types¶
You are deciding whether to eat at Dirty Birds or The Loft.
Suppose Yelp shows ratings aggregated by phone type (Android vs. iPhone).
Phone Type | Stars for Dirty Birds | Stars for The Loft |
---|---|---|
Android | 4.24 | 4.0 |
iPhone | 2.99 | 2.79 |
All | 3.32 | 3.37 |
Question: Should you choose Dirty Birds or The Loft?
Answer: The type of phone you use likely has nothing to do with your taste in food – pick the restaurant that is rated higher overall.
Remember, Simpson's paradox is merely a property of weighted averages!
Takeaways¶
Be skeptical of...
- Aggregate statistics.
- People misusing statistics to "prove" that discrimination doesn't exist.
- Drawing conclusions from individual publications ($p$-hacking, publication bias, narrow focus, etc.).
- Everything!
We need to apply domain knowledge and human judgement calls to decide what to do when Simpson's paradox is present.
Really?¶
To handle Simpson's paradox with rigor, we need some ideas from causal inference which we don't have time to cover in DSC 80. This video has a good example of how to approach Simpson's paradox, using a minimal amount of causal inference, if you're curious (not required for DSC 80).
IFrame('https://www.youtube-nocookie.com/embed/zeuW1Z2EtLs?si=l2Dl7P-5RCq3ODpo',
width=560, height=315)
Further reading¶
- Gender Bias in Admission Statistics?
- Contains a great visualization, but seems to be paywalled now.
- What is Simpson's Paradox?
Summary, next time¶
- Grouping allows us to change the level of granularity in a DataFrame.
- Grouping involves three steps – split, apply, and combine (or filter, or transform).
pivot_table
aggregates data based on two categorical columns, and reshapes the result to be "wide" instead of "long".- Simpson's paradox occurs when grouped data and ungrouped data show opposing trends.
- It is a consequence of arithmetic.
- Next time: Data cleaning! 🧼