from dsc80_utils import *
Announcements 📣¶
Guest lecture on Thursday Dec 5, 1:30pm-3pm in the HDSI MPR: Dr. Mohammad Ramezanali, an AI lead from Salesforce, will be talking about LLMs and how he uses them in industry.
- No regular lecture on Dec 5.
- If you attend the guest lecture, you will get lecture attendance credit and 1% extra credit on your final exam grade.
- If you can't make it, we'll record the talk and you can get attendance + extra credit by making a post on Ed with a few paragraphs about the talk (details to come).
The Final Project is due on Tue Dec 10.
- No slip days allowed!
The Final Exam is on Saturday, Dec 7 from 11:30am-2:30pm in PODEM 1A18 and 1A19.
If at least 80% of the class fills out both SETs and the End-of-Quarter Survey by Friday, Dec 6 at 11:59PM, then everyone will earn an extra 1% on the Final Exam.
Model fairness¶
Fairness: why do we care?¶
- Sometimes, a model performs better for certain groups than others; in such cases we say the model is unfair.
- Since ML models are now used in processes that significantly affect human lives, it is important that they are fair!
- Job applications and college admissions.
- Criminal sentencing and parole grants.
- Predictive policing.
- Credit and loans.
Model fairness¶
- We'd like to build a model that is fair, meaning that it performs the same for individuals within a group and individuals outside of the group.
- What do we mean by "perform"? What do we mean by "the same"?
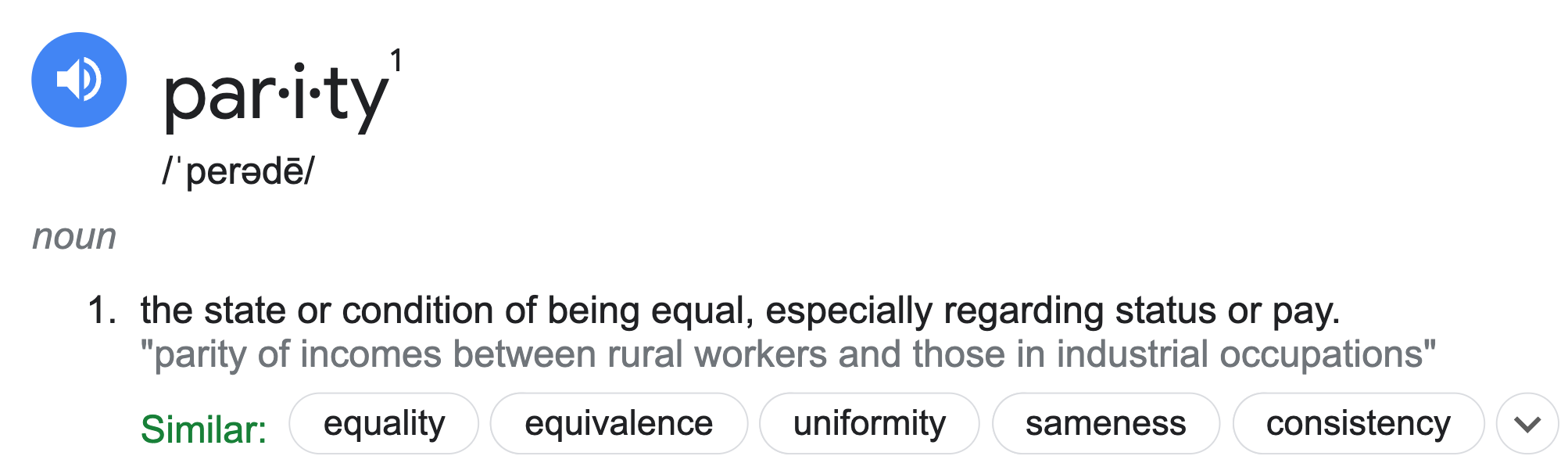
Parity measures for classifiers¶
Suppose $C$ is a classifier we've already trained, and $A$ is some binary attribute that denotes whether an individual is a member of a sensitive group – that is, a group we want to avoid discrimination for (e.g. $A = \text{age is less than 25}$).
- $C$ achieves accuracy parity if $C$ has the same accuracy for individuals in $A$ and individuals not in $A$.
- Example: $C$ is a binary classifier that determines whether someone receives a loan.
- If the classifier predicts correctly, then either $C$ approves the loan and it is paid off, or $C$ denies the loan and it would have defaulted.
- If $C$ achieves accuracy parity, then the proportion of correctly classified loans should be the same for those under 25 and those over 25.
- Example: $C$ is a binary classifier that determines whether someone receives a loan.
- $C$ achieves precision (or recall) parity if $C$ has the same precision (or recall) for individuals in $A$ and individuals not in $A$.
- Recall parity is often called "true positive rate parity."
- $C$ achieves demographic parity if the proportion of predictions that are positive is equal for individuals in $A$ and individuals not in $A$.
- With the exception of demographic parity, the parity measures above all involve checking whether some evaluation metric from Lecture 17 is equal across two groups.
More on parity measures¶
- Which parity metric should you care about? It depends on your specific dataset and what types of errors are important!
- Many of these parity measures are impossible to satisfy simultaneously!
- The classifier parity metrics mentioned on the previous slide are only a few of the many possible parity metrics. See these DSC 167 notes for more details, including more formal explanations.
- These don't apply for regression models; for those, we may care about RMSE parity or $R^2$ parity. There is also a notion of demographic parity for regression models, but it is outside of the scope of DSC 80.
Example: Loan approval¶
As you know from Project 2, LendingClub was a "peer-to-peer lending company"; they used to publish a dataset describing the loans that they approved.
'tag'
: whether loan was repaid in full (1.0) or defaulted (0.0).'loan_amnt'
: amount of the loan in dollars.'emp_length'
: number of years employed.'home_ownership'
: whether borrower owns (1.0) or rents (0.0).'inq_last_6mths'
: number of credit inquiries in last six months.'revol_bal'
: revolving balance on borrows accounts.'age'
: age in years of the borrower (protected attribute).
loans = pd.read_csv(Path('data') / 'loan_vars1.csv', index_col=0)
loans.head()
loan_amnt | emp_length | home_ownership | inq_last_6mths | revol_bal | age | tag | |
---|---|---|---|---|---|---|---|
268309 | 6400.0 | 0.0 | 1.0 | 1.0 | 899.0 | 22.0 | 0.0 |
301093 | 10700.0 | 10.0 | 1.0 | 0.0 | 29411.0 | 19.0 | 0.0 |
1379211 | 15000.0 | 10.0 | 1.0 | 2.0 | 9911.0 | 48.0 | 0.0 |
486795 | 15000.0 | 10.0 | 1.0 | 2.0 | 15883.0 | 35.0 | 0.0 |
1481134 | 22775.0 | 3.0 | 1.0 | 0.0 | 17008.0 | 39.0 | 0.0 |
The total amount of money loaned was over 5 billion dollars!
loans['loan_amnt'].sum()
np.float64(5706507225.0)
loans.shape[0]
386772
Predicting 'tag'
¶
Let's build a classifier that predicts whether or not a loan was paid in full. If we were a bank, we could use our trained classifier to determine whether to approve someone for a loan!
from sklearn.model_selection import train_test_split
from sklearn.ensemble import RandomForestClassifier
X = loans.drop('tag', axis=1)
y = loans.tag
X_train, X_test, y_train, y_test = train_test_split(X, y)
clf = RandomForestClassifier(n_estimators=50)
clf.fit(X_train, y_train)
RandomForestClassifier(n_estimators=50)In a Jupyter environment, please rerun this cell to show the HTML representation or trust the notebook.
On GitHub, the HTML representation is unable to render, please try loading this page with nbviewer.org.
RandomForestClassifier(n_estimators=50)
Recall, a prediction of 1 means that we predict that the loan will be paid in full.
y_pred = clf.predict(X_test)
y_pred
array([0., 1., 1., ..., 1., 1., 0.])
clf.score(X_test, y_test)
0.7129471626694797
from sklearn.metrics import ConfusionMatrixDisplay
ConfusionMatrixDisplay.from_estimator(clf, X_test, y_test);
plt.grid(False)
Precision¶
$$\text{precision} = \frac{TP}{TP+FP}$$
Precision describes the proportion of loans that were approved that would have been paid back.
from sklearn import metrics
metrics.precision_score(y_test, y_pred)
np.float64(0.7715641199421172)
If we subtract the precision from 1, we get the proportion of loans that were approved that would not have been paid back. This is known as the false discovery rate.
$$\frac{FP}{TP + FP} = 1 - \text{precision}$$
1 - metrics.precision_score(y_test, y_pred)
np.float64(0.22843588005788285)
Recall¶
$$\text{recall} = \frac{TP}{TP + FN}$$
Recall describes the proportion of loans that would have been paid back that were actually approved.
metrics.recall_score(y_test, y_pred)
np.float64(0.7337432717264445)
If we subtract the recall from 1, we get the proportion of loans that would have been paid back that were denied. This is known as the false negative rate.
$$\frac{FN}{TP + FN} = 1 - \text{recall}$$
1 - metrics.recall_score(y_test, y_pred)
np.float64(0.2662567282735555)
From both the perspective of the bank and the lendee, a high false negative rate is bad!
- The bank left money on the table – the lendee would have paid back the loan, but they weren't approved for a loan.
- The lendee deserved the loan, but weren't given one.
False negative rate by age¶
results = X_test
results['age_bracket'] = results['age'].apply(lambda x: 5 * (x // 5 + 1))
results['prediction'] = y_pred
results['tag'] = y_test
(
results
.groupby('age_bracket')
[['tag', 'prediction']]
.apply(lambda x: 1 - metrics.recall_score(x['tag'], x['prediction']))
.plot(kind='bar', title='False Negative Rate by Age Group')
)
Computing parity measures¶
- $C$: Our random forest classifier (1 if we approved the loan, 0 if we denied it).
- $A$: Whether or not they were under 25 (1 if under, 0 if above).
results['is_young'] = (results['age'] < 25).replace({True: 'young', False: 'old'})
First, let's compute the proportion of loans that were approved in each group. If these two numbers are the same, $C$ achieves demographic parity.
results.groupby('is_young')['prediction'].mean()
is_young old 0.69 young 0.30 Name: prediction, dtype: float64
$C$ evidently does not achieve demographic parity – older people are approved for loans far more often! Note that this doesn't factor in whether they were correctly approved or incorrectly approved.
Now, let's compute the accuracy of $C$ in each group. If these two numbers are the same, $C$ achieves accuracy parity.
compute_accuracy = lambda x: metrics.accuracy_score(x['tag'], x['prediction'])
(
results
.groupby('is_young')
[['tag', 'prediction']]
.apply(compute_accuracy)
.rename('accuracy')
)
is_young old 0.73 young 0.67 Name: accuracy, dtype: float64
Hmm... These numbers look much more similar than before!
Is this difference in accuracy significant?¶
Let's run a permutation test to see if the difference in accuracy is significant.
- Null Hypothesis: The classifier's accuracy is the same for both young people and old people, and any differences are due to chance.
- Alternative Hypothesis: The classifier's accuracy is higher for old people.
- Test statistic: Difference in accuracy (young minus old).
- Significance level: 0.01.
obs = (results
.groupby('is_young')
[['tag', 'prediction']]
.apply(compute_accuracy)
.diff()
.iloc[-1])
obs
np.float64(-0.057039394708304325)
diff_in_acc = []
for _ in range(500):
s = (
results[['is_young', 'prediction', 'tag']]
.assign(is_young=np.random.permutation(results['is_young']))
.groupby('is_young')
[['tag', 'prediction']]
.apply(compute_accuracy)
.diff()
.iloc[-1]
)
diff_in_acc.append(s)
fig = pd.Series(diff_in_acc).plot(kind='hist', histnorm='probability', nbins=20,
title='Difference in Accuracy (Young - Old)')
fig.add_vline(x=obs, line_color='red')
fig.update_layout(xaxis_range=[-0.1, 0.05])
It seems like the difference in accuracy across the two groups is significant, despite being only ~5%. Thus, $C$ likely does not achieve accuracy parity.
Ethical questions of fairness¶
Question 🤔 (Answer at dsc80.com/q)
Code: fair
- Question: Is it "fair" to deny loans to younger people at a higher rate?
- Make an argument for "yes", then make an argument for "no".
- Federal law prevents age from being used as a determining factor in denying a loan.
Not only should we use 'age'
to determine whether or not to approve a loan, but we also shouldn't use other features that are strongly correlated with 'age'
, like 'emp_length'
.
loans
loan_amnt | emp_length | home_ownership | inq_last_6mths | revol_bal | age | tag | |
---|---|---|---|---|---|---|---|
268309 | 6400.0 | 0.0 | 1.0 | 1.0 | 899.0 | 22.0 | 0.0 |
301093 | 10700.0 | 10.0 | 1.0 | 0.0 | 29411.0 | 19.0 | 0.0 |
1379211 | 15000.0 | 10.0 | 1.0 | 2.0 | 9911.0 | 48.0 | 0.0 |
... | ... | ... | ... | ... | ... | ... | ... |
1150493 | 5000.0 | 1.0 | 1.0 | 0.0 | 3842.0 | 52.0 | 1.0 |
686485 | 6000.0 | 10.0 | 0.0 | 0.0 | 6529.0 | 36.0 | 1.0 |
342901 | 15000.0 | 8.0 | 1.0 | 1.0 | 16060.0 | 39.0 | 1.0 |
386772 rows × 7 columns
Parting Thoughts¶
Course goals ✅¶
In this course, you...
- Practiced translating potentially vague questions into quantitative questions about measurable observations.
- Learned to reason about 'black-box' processes (e.g. complicated models).
- Understood computational and statistical implications of working with data.
- Learned to use real data tools (e.g. love the documentation!).
- Got a taste of the "life of a data scientist".
Course outcomes ✅¶
Now, you...
- Are prepared for internships and data science "take home" interviews!
- Are ready to create your own portfolio of personal projects.
- Have the background and maturity to succeed in the upper-division.
Topics covered ✅¶
We learnt a lot this quarter.
- Week 1: From BabyPandas to Pandas
- Week 2: DataFrames
- Week 3: Messy Data, Hypothesis Testing
- Week 4: Missing Values and Imputation
- Week 5: HTTP, Midterm Exam
- Week 6: Web Scraping, Regex
- Week 7: Text Features, Regression
- Week 8: Feature Engineering
- Week 9: Generalization, CV, Decision Trees
- Week 10: Random Forests, Classifier Evaluation
Thank you!¶
This course would not have been possible without our 11 tutors and 1 TA: Mizuho Fukada, Gabriel Cha, Anish Kasam, Ylesia Wu, Sunan Xu, Andrew Yang, Luran (Lauren) Zhang, and Qirui (Sara) Zheng.
Don't be a stranger – our contact information is at dsc80.com/staff!
- This quarter's course website will remain online permanently at dsc-courses.github.io.
Apply to be a tutor in the future! Learn more here.
Building a Data Science Career¶
Do Grades Matter?¶
- Not as much as you probably think.
What you might imagine:
- Student works hard to get As in their DSC classes.
- High GPA looks good on resume.
- Resume leads to interview, student aces interview.
- Good job!
But this is what I actually see:
- Student works hard to get As in ALL OF THEIR classes and takes a minor / double major.
- High GPA looks decent on resume, but lots of students have high GPAs.
- Resume doesn't stand out very much, and student has trouble finding job after graduation.
An Alternate Route¶
- Student takes fewer classes in order to do AMAZING work in one class (e.g. really interested in data visualization).
- Professor: Wow, that was the best class work in YEARS, you should totally join my research group!
- Student works with PhD students and professor on cutting-edge research, years ahead of what's available in industry.
- Student's resume is packed with interesting projects that VERY FEW other students have, leading to an AWESOME JOB after graduation.
- Or, student applies to grad school and their professor can write them a super good letter.
There are many ways to be excellent¶
- Take more classes, get good grades
- Highly structured -- professor gives you problems.
Less-structured efforts where you pick the problem to work on:
- Go beyond the requirements in a course you really like.
- Help redesign a course.
- Conduct an independent study.
- Do research.
- Create a startup / non-profit.
Higher risk of failure, but you'll learn a lot more than if you just take classes!
An Email¶
Hey Sam, I'm glad you're talking about this. When I look at resumes, I skip past the GPA and courses and look at their project-based courses and what they did in those courses. Bonus points if they have independent projects, and if there's a link to their project on their resume, I will always click on it.
My Advice for People Starting Out¶
- Next quarter, take fewer courses, and be okay with getting Bs in some courses if it means you get to invest lots of time into the one course you really like.
- Really stand out in one course you like. Here are some examples:
- Going 2x above project requirements, then talk to your professor about your work.
- Ask lots of good questions after every lecture.
- Come to office hours regularly with questions about topics beyond the course.
- At the end of the quarter, ask your professor if you can help with their research group.
In Other Words...¶
There are "grades" you can get that are (much, much) higher than A's!
(From Dave Eckhardt)
Let's Be Pragmatic¶
- Yes, you need to work hard to develop strong technical skills!
- But the best jobs come through people that know you, not by submitting your resume into a pool of thousands of applicants.
- You should spend time thinking about how you can make use of being at UCSD to grow your network.
- PhD students and professors are a good place to start, but not the only way.
How to cold-email and actually get responses¶
- Pick a topic of interest.
- Do an independent project around that topic.
- Small and punchy is better than big! See some of Simon Willison's posts for examples.
- Put your project online as a publicly viewable webpage (like what you're doing for your final project!).
- Write an email that looks like this:
Hi {name},
My name is {name} and I'm an undergrad at UCSD studying {major}.
I'm really interested in learning more about your research in {topic} since I'm also working on projects in that area. For example, I wrote about my latest work on {my project} here: {url}.
Would you have some time in the next few weeks for a 30-min chat?
Key points:
- Email PhD students, not professors!
- Most professors I know get lots of low-quality cold emails every day, so many of them just ignore. But PhD students are less famous so they'll be happy that someone is interested in their work.
- Keep it short. If they can't read it in 10 seconds, it's too long!
- Share your URL. That shows that you actually have interest in the topic.
- Ask for a 30-min chat (not 1 hour). Again, people are busy!
- If they agree to meet, don't ask to join their lab right away. Talk to them about their work, then at the end, say something like, "Thanks again for taking the time to meet with me! This work fits really well with my interests, so I was wondering whether there might be opportunities to work with you as part of your research group."
- If they say yes, great! If they say no, ask them for other people they know who might also be a good fit.
Shifting your mentality¶
- What you want to avoid:
- "Please give me a research position, I'll do anything."
- Even though this might be truly how you feel!
- What you want to think instead:
- I'm a rising professional with lots of highly valuable skills.
- I also have specific interests.
- I'm looking for the right position that aligns with my interests so that I can contribute in a productive and meaningful way.
Career Advice from Kelly Jensen¶
Kelly will come and present.
Final Exam Review¶
Let's review for the final!
Course Topics¶
- Working with pandas
- Exploring and cleaning data
- Hypothesis and permutation testing
- Missingness and imputation
- HTTP and HTML
- Regular expressions
- Text features
- Linear regression
- Feature engineering
- Generalization and cross-validation
- Decision trees
- Classifier evaluation and fairness
Question 🤔 (Answer at dsc80.com/q)
Code: review
Submit one question from a past exam that you want to review. Copy-paste this format:
URL:
Question:
Topic:
For example, if you want to review question 1 from SP24's final, you would enter in:
URL: https://practice.dsc80.com/sp24-final/index.html
Question: Q1
Topic: pandas