from dsc80_utils import *
# For the JSON evaluation example.
def err():
raise ValueError('i just deleted all your files lol 😂')
Announcements 📣¶
- Project 2's checkpoint is due today. The full project is due on Tuesday, February 13th.
- You can use up to 3 slip days on both deadlines, and everyone now has 7 slip days instead of 6.
- Lab 5 is due on Monday, February 12th.
- It doesn't have any hidden tests, since it's due close to the Midterm Exam.
- It has lots of conceptual problems on missingness mechanisms, so try and work through it before the exam!
- The Midterm Exam is this Thursday in lecture.
Midterm Exam 📝¶
Thursday, February 8th, from 3:30-4:50PM, in Pepper Canyon 109
- Pen and paper only. No calculators, phones, or watches allowed.
- You will be assigned a seat by tomorrow!
- You are allowed to bring one double-sided 8.5" x 11" sheet of handwritten notes.
- No reference sheet given, unlike DSC 10!
- We will display clarifications and the time remaining during the exam.
- Covers Lectures 1-8 and all related assignments.
- To review problems from old exams, go to practice.dsc80.com.
- We just posted the Fall 2023 Midterm and solutions.
- Also look at the Resources tab on the course website.
Agenda 📆¶
- Introduction to HTTP.
- Making HTTP requests.
- Data formats.
- APIs and web scraping.
- Midterm review.
Introduction to HTTP¶
Data sources¶
Often, the data you need doesn't exist in "clean"
.csv
files.Solution: Collect your own data!
- Design and administer your own survey or run an experiment.
- Find related data on the internet.
- The internet contains massive amounts of historical record; for most questions you can think of, the answer exists somewhere on the internet.
Collecting data from the internet¶
There are two ways to programmatically access data on the internet:
- through an API.
- by scraping.
We will discuss the differences between both approaches, but for now, the important part is that they both use HTTP.
HTTP¶
HTTP stands for Hypertext Transfer Protocol.
- It was developed in 1989 by Tim Berners-Lee (and friends).
It is a request-response protocol.
- Protocol = set of rules.
HTTP allows...
- computers to talk to each other over a network.
- devices to fetch data from "web servers."
The "S" in HTTPS stands for "secure".
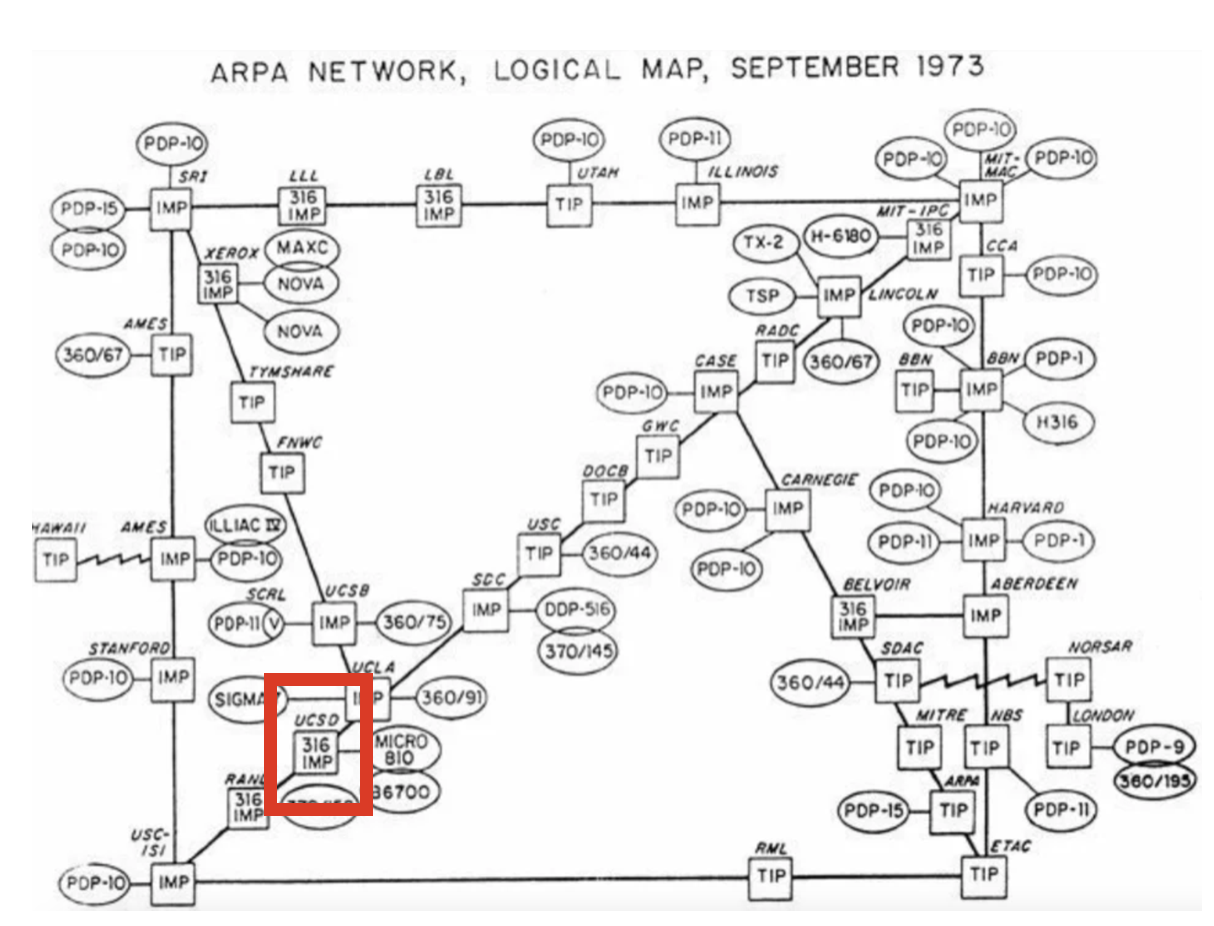
UCSD was a node in ARPANET, the predecessor to the modern internet (source).
The request-response model¶
HTTP follows the request-response model.
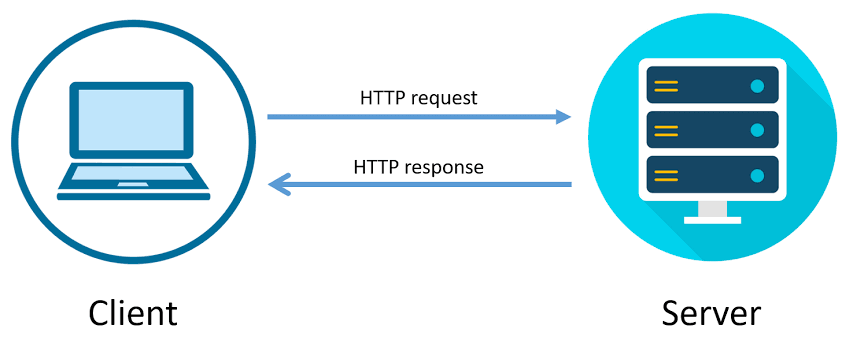
A request is made by the client.
A response is returned by the server.
Example: YouTube search 🎥.
- Consider the following URL: https://www.youtube.com/results?search_query=apple+vision+pro.
- Your web browser, a client, makes an HTTP request with a search query.
- The server, YouTube, is a computer that is sitting somewhere else.
- The server returns a response that contains the search results.
- Note: ?search_query=apple+vision+pro is called a "query string."
Request methods¶
The request methods you will use most often are GET
and POST
; see Mozilla's web docs for a detailed list of request methods.
GET
is used to request data from a specified resource.POST
is used to send data to the server.- For example, uploading a photo to Instagram or entering credit card information on Amazon.
Example GET
request¶
Below is an example GET
HTTP request made by a browser when accessing datascience.ucsd.edu.
GET / HTTP/1.1
Connection: keep-alive
Host: datascience.ucsd.edu
User-Agent: Mozilla/5.0 (Macintosh; Intel Mac OS X 10_15_7) AppleWebKit/537.36 (KHTML, like Gecko) Chrome/121.0.0.0 Safari/537.36
sec-ch-ua: "Chromium";v="121", "Not A(Brand";v="99"
sec-ch-ua-platform: "macOS"
The first line (
GET / HTTP/1.1
) is called the "request line", and the lines afterwards are called "header fields". Header fields contain metadata.We could also provide a "body" after the header fields.
To see HTTP requests in Google Chrome, follow these steps.
Example GET
response¶
The response below was generated by executing the request on the previous slide.
HTTP/1.1 200 OK
Date: Sun, 04 Feb 2024 17:35:01 GMT
Server: Apache
X-Powered-By: PHP/7.4.33
Link: <https://datascience.ucsd.edu/wp-json/>; rel="https://api.w.org/"
Link: <https://datascience.ucsd.edu/wp-json/wp/v2/pages/113>; rel="alternate"; type="application/json"
...
<html lang="en-US">
<head>
<meta charset="UTF-8">
<meta name="viewport" content="width=device-width, initial-scale=1.0"/>
<meta http-equiv="X-UA-Compatible" content="IE=edge">
<link rel="profile" href="https://gmpg.org/xfn/11"/>
<title>Halıcıoğlu Data Science Institute –UC San Diego</title>
<script>
...
Consequences of the request-response model¶
When a request is sent to view content on a webpage, the server must:
- process your request (i.e. prepare data for the response).
- send content back to the client in its response.
Remember, servers are computers.
- Someone has to pay to keep these computers running.
- This means that every time you access a website, someone has to pay.
Making HTTP requests¶
Making HTTP requests¶
There are (at least) two ways to make HTTP requests outside of a browser:
From the command line, with
curl
.From Python, with the
requests
package.
Making HTTP requests using requests
¶
requests
is a Python module that allows you to use Python to interact with the internet!- There are other packages that work similarly (e.g.
urllib
), butrequests
is arguably the easiest to use.
import requests
Example: GET
requests via requests
¶
For instance, let's access the source code of the UCSD homepage, https://ucsd.edu.
res = requests.get('https://ucsd.edu')
res
is now a Response
object.
res
<Response [200]>
The text
attribute of res
is a string that containing the entire response.
type(res.text)
str
len(res.text)
45296
print(res.text[:1000])
<!DOCTYPE html> <html lang="en"> <head> <meta charset="utf-8"/> <meta content="IE=edge" http-equiv="X-UA-Compatible"/> <meta content="width=device-width, initial-scale=1" name="viewport"/> <title>University of California San Diego</title> <meta content="University of California, San Diego" name="ORGANIZATION"/> <meta content="index,follow,noarchive" name="robots"/> <meta content="UCSD" name="SITE"/> <meta content="University of California San Diego" name="PAGETITLE"/> <meta content="The University California San Diego is one of the world's leading public research universities, located in beautiful La Jolla, California" name="DESCRIPTION"/> <link href="favicon.ico" rel="icon"/> <!-- Site-specific CSS files --> <link href="https://www.ucsd.edu/_resources/css/vendor/brix_sans.css" rel="stylesheet" type="text/css"/> <link href="https://www.ucsd.edu/_resources/css/vendor/refrigerator_deluxe.css" rel="stylesheet"
Example: POST
requests via requests
¶
The following call to requests.post
makes a post request to https://httpbin.org/post, with a 'name'
parameter of 'King Triton'
.
post_res = requests.post('https://httpbin.org/post',
data={'name': 'King Triton'})
post_res
<Response [200]>
print(post_res.text)
{ "args": {}, "data": "", "files": {}, "form": { "name": "King Triton" }, "headers": { "Accept": "*/*", "Accept-Encoding": "gzip, deflate, br", "Content-Length": "16", "Content-Type": "application/x-www-form-urlencoded", "Host": "httpbin.org", "User-Agent": "python-requests/2.31.0", "X-Amzn-Trace-Id": "Root=1-65c2f3d4-1846efc848c3019151e00d53" }, "json": null, "origin": "70.95.172.151", "url": "https://httpbin.org/post" }
# More on this shortly!
post_res.json()
{'args': {}, 'data': '', 'files': {}, 'form': {'name': 'King Triton'}, 'headers': {'Accept': '*/*', 'Accept-Encoding': 'gzip, deflate, br', 'Content-Length': '16', 'Content-Type': 'application/x-www-form-urlencoded', 'Host': 'httpbin.org', 'User-Agent': 'python-requests/2.31.0', 'X-Amzn-Trace-Id': 'Root=1-65c2f3d4-1846efc848c3019151e00d53'}, 'json': None, 'origin': '70.95.172.151', 'url': 'https://httpbin.org/post'}
What happens when we try and make a POST
request somewhere where we're unable to?
yt_res = requests.post('https://youtube.com',
data={'name': 'King Triton'})
yt_res
<Response [400]>
yt_res.text
'<html lang="en" dir="ltr"><head><title>Oops</title><style nonce="4UnFgFL8ztc32AfxDCUwbQ">html{font-family:Roboto,Arial,sans-serif;font-size:14px}body{background-color:#f9f9f9;margin:0}#content{max-width:440px;margin:128px auto}svg{display:block;pointer-events:none}#monkey{width:280px;margin:0 auto}h1,p{text-align:center;margin:0;color:#131313}h1{padding:24px 0 8px;font-size:24px;font-weight:400}p{line-height:21px}sentinel{}</style><link rel="shortcut icon" href="https://www.youtube.com/img/favicon.ico" type="image/x-icon"><link rel="icon" href="https://www.youtube.com/img/favicon_32.png" sizes="32x32"><link rel="icon" href="https://www.youtube.com/img/favicon_48.png" sizes="48x48"><link rel="icon" href="https://www.youtube.com/img/favicon_96.png" sizes="96x96"><link rel="icon" href="https://www.youtube.com/img/favicon_144.png" sizes="144x144"></head><body><div id="content"><h1>Something went wrong</h1><p><svg id="monkey" viewBox="0 0 490 525"><path fill="#6A1B9A" d="M325 85c1 12-1 25-5 38-8 29-31 52-60 61-26 8-54 14-81 18-37 6-26-37-38-72l-4-4c0-17-9-33 4-37l33-4c9-2 9-21 11-30 1-7 3-14 5-21 8-28 40-42 68-29 18 9 36 19 50 32 13 11 16 31 17 48z"/><path fill="none" stroke="#6A1B9A" stroke-width="24" stroke-linecap="round" stroke-miterlimit="10" d="M431 232c3 15 21 19 34 11 15-9 14-30 5-43-12-17-38-25-59-10-23 18-27 53-21 97s1 92-63 108"/><path fill="#6A1B9A" d="M284 158c35 40 63 85 86 133 24 52-6 113-62 123-2 0-4 1-6 1-53 9-101-33-101-87V188l83-30z"/><path fill="#F7CB4D" d="M95 152c-3-24 13-57 46-64l27-5c9-2 16-19 17-28l3-15 20-3c44 14 42 55 18 69 22 0 39 26 32 53-5 18-20 32-39 36-13 3-26 5-40 8-50 8-80-14-84-51z"/><path fill="#6A1B9A" d="M367 392c-21 18-77 70-25 119h-61c-27-29-32-69 1-111l85-8z"/><path fill="#6A1B9A" d="M289 399c-21 18-84 62-32 111h-61c-37-34-5-104 43-134l50 23z"/><path fill="#EDB526" d="M185 56l3-15 20-3c25 8 35 25 35 41-12-18-49-29-58-23z"/><path fill="#E62117" d="M190 34c8-28 40-42 68-29 18 9 36 19 50 32 10 9 14 23 16 37L187 46l3-12z"/><path fill="#8E24AA" d="M292 168c0 0 0 201 0 241s20 98 91 85l-16-54c-22 12-31-17-31-37 0-20 0-108 0-137S325 200 292 168z"/><path fill="#F7CB4D" d="M284 79c11-9 23-17 35-23 25-12 54 7 59 38v1c4 27-13 51-36 53-12 1-25 1-37 0-22-1-39-27-32-52v-1c2-6 6-12 11-16z"/><path fill="#6A1B9A" d="M201 203s0 84-95 140l22 42s67-25 89-86-16-96-16-96z"/><path fill="#BE2117" d="M224 54l-67-14c-10-2-13-15-5-21s18-6 26 0l46 35z"/><circle fill="#4A148C" cx="129" cy="161" r="12"/><circle fill="#4A148C" cx="212" cy="83" r="7"/><circle fill="#4A148C" cx="189" cy="79" r="7"/><path fill="#F7CB4D" d="M383 493c11-3 19-8 25-13 7-10 4-16-5-20 8-9 2-22-8-18 1-1 1-2 1-3 3-9-9-15-15-8-3 4-8 7-13 9l15 53z"/><path fill="#EDB526" d="M252 510c5 6 0 15-9 15h-87c-10 0-16-8-13-15 5-12 21-19 36-16l73 16z"/><ellipse transform="rotate(19.126 278.35 14.787)" fill="#E62117" cx="278" cy="15" rx="9" ry="7"/><path fill="#F7CB4D" d="M341 510c5 6 0 15-9 15h-87c-10 0-16-8-13-15 5-12 21-19 36-16l73 16z"/><path fill="#EDB526" d="M357 90c-12-19-35-23-55-11-19 12-25 32-13 52"/><path fill="#E62117" d="M110 427l21-9c5-2 7-8 5-13l-42-94c-3-6-9-9-15-6l-11 5c-6 2-9 9-7 15l36 97c2 5 8 7 13 5z"/><path fill="#B0BEC5" d="M37 278l41-17c11-4 22-5 33-1 5 2 10 4 14 6 6 3 4 11-3 11-9 0-18 1-26 3l2 12c1 6-2 11-8 13l-36 15c-5 2-10 1-14-2l-9-7-2 17c0 2-2 4-4 5l-3 1c-3 1-7 0-8-3L1 300c-1-3 0-7 4-9l4-2c2-1 5 0 7 1l12 10 1-11c0-5 3-9 8-11z"/><path fill="#F7CB4D" d="M103 373c10 2 14 10 8 19 6-1 10 4 10 9 0 3-3 6-6 7l-26 11c-2 1-5 1-8 0-6-3-7-9-2-16-7-1-13-9-6-17-8-1-12-8-8-15l3-3 23-11c9-4 19 8 12 16z"/><ellipse transform="rotate(173.3 233.455 334.51)" fill="#8E24AA" cx="234" cy="335" rx="32" ry="46"/></svg></p><style nonce="4UnFgFL8ztc32AfxDCUwbQ">#yt-masthead{margin:15px auto;width:440px;margin-top:25px}#logo-container{margin-right:5px;float:left;cursor:pointer;text-decoration:none}.logo{background:no-repeat url(//www.gstatic.com/youtube/img/branding/youtubelogo/1x/youtubelogo_30.png);width:125px;height:30px;cursor:pointer;display:inline-block}#masthead-search{display:-webkit-box;display:-webkit-flex;display:flex;margin-top:3px;max-width:650px;overflow:hidden;padding:0;position:relative}.search-button{border-left:0;border-top-left-radius:0;border-bottom-left-radius:0;float:right;height:29px;padding:0;border:solid 1px transparent;border-color:#d3d3d3;background:#f8f8f8;color:#333;cursor:pointer}.search-button:hover{border-color:#c6c6c6;background:#f0f0f0;-webkit-box-shadow:0 1px 0 rgba(0,0,0,.1);box-shadow:0 1px 0 rgba(0,0,0,.1)}.search-button-content{border:none;display:block;opacity:.6;padding:0;text-indent:-10000px;background:no-repeat url(//www.gstatic.com/youtube/src/web/htdocs/img/search.png);-webkit-background-size:auto auto;background-size:auto;width:15px;height:15px;-webkit-box-shadow:none;box-shadow:none;margin:0 25px}#masthead-search-terms-border{-webkit-box-flex:1;-webkit-flex:1 1 auto;flex:1 1 auto;border:1px solid #ccc;-webkit-box-shadow:inset 0 1px 2px #eee;box-shadow:inset 0 1px 2px #eee;background-color:#fff;font-size:14px;height:29px;line-height:30px;margin:0 0 2px;overflow:hidden;position:relative;-webkit-box-sizing:border-box;box-sizing:border-box;-webkit-transition:border-color .2s ease;transition:border-color .2s ease}#masthead-search-terms{background:transparent;border:0;font-size:16px;height:100%;left:0;margin:0;outline:none;padding:2px 6px;position:absolute;width:100%;-webkit-box-sizing:border-box;box-sizing:border-box}sentinel{}</style><div id="yt-masthead"><a id="logo-container" href="https://www.youtube.com/" title="YouTube home"><span class="logo" title="YouTube home"></span></a><form id="masthead-search" class="search-form" action="https://www.youtube.com/results"><script nonce="Vm3374R_T0bfzfjvIJ-Dtg">document.addEventListener(\'DOMContentLoaded\', function () {document.getElementById(\'masthead-search\').addEventListener(\'submit\', function(e) {if (document.getElementById(\'masthead-search-terms\').value == \'\') {e.preventDefault();}});});</script><div id="masthead-search-terms-border" dir="ltr"><input id="masthead-search-terms" autocomplete="off" name="search_query" value="" type="text" placeholder="Search" title="Search" aria-label="Search"><script nonce="Vm3374R_T0bfzfjvIJ-Dtg">document.addEventListener(\'DOMContentLoaded\', function () {document.getElementById(\'masthead-search-terms\').addEventListener(\'keydown\', function() {if (!this.value && (event.keyCode == 40 || event.keyCode == 32 || event.keyCode == 34)) {this.onkeydown = null; this.blur();}});});</script></div><button id="masthead-search-button" class="search-button" type="submit" dir="ltr"><script nonce="Vm3374R_T0bfzfjvIJ-Dtg">document.addEventListener(\'DOMContentLoaded\', function () {document.getElementById(\'masthead-search-button\').addEventListener(\'click\', function(e) {if (document.getElementById(\'masthead-search-terms\').value == \'\') {e.preventDefault(); return;}e.preventDefault(); document.getElementById(\'masthead-search\').submit();});});</script><span class="search-button-content">Search</span></button></form></div></div></body></html>'
yt_res.text
is a string containing HTML – we can render this in-line using IPython.display.HTML
.
from IPython.display import HTML
HTML(yt_res.text)
HTTP status codes¶
- When we request data from a website, the server includes an HTTP status code in the response.
- The most common status code is
200
, which means there were no issues.
- Other times, you will see a different status code, describing some sort of event or error.
- Common examples:
400
– bad request,404
– page not found,500
– internal server error. - The first digit of a status describes its general "category".
- Common examples:
- See https://httpstat.us for a list of all HTTP status codes.
- It also has example sites for each status code; for example, https://httpstat.us/404 returns a
404
.
- It also has example sites for each status code; for example, https://httpstat.us/404 returns a
yt_res.status_code
400
# ok checks if the result was successful.
yt_res.ok
False
Handling unsuccessful requests¶
Unsuccessful requests can be re-tried, depending on the issue.
- A good first step is to wait a little, then try again.
A common issue is that you're making too many requests to a particular server at a time – if this is the case, increase the time between each request. You can even do this programatically, say, using
time.sleep
.See the textbook for more examples.
Data formats¶
The data formats of the internet¶
Responses typically come in one of two formats: HTML or JSON.
The response body of a
GET
request is usually either JSON (when using an API) or HTML (when accessing a webpage).The response body of a
POST
request is usually JSON.XML is also a common format, but not as popular as it once was.
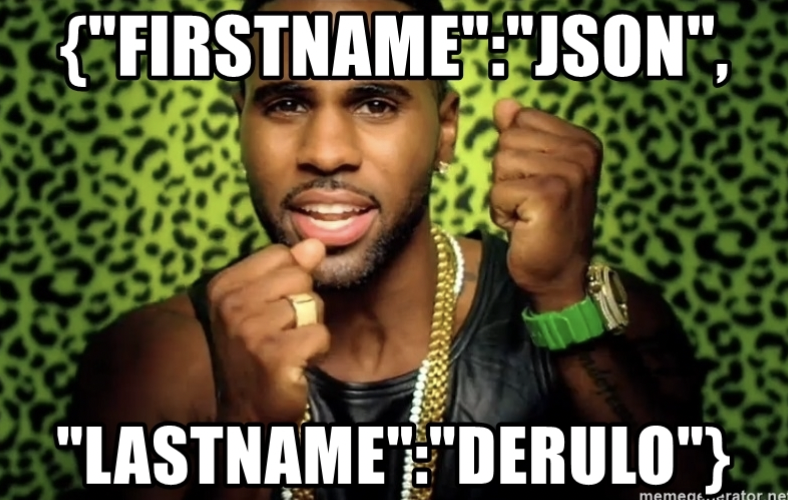
JSON¶
JSON stands for JavaScript Object Notation. It is a lightweight format for storing and transferring data.
It is:
- very easy for computers to read and write.
- moderately easy for programmers to read and write by hand.
- meant to be generated and parsed.
Most modern languages have an interface for working with JSON objects.
- JSON objects resemble Python dictionaries (but are not the same!).
JSON data types¶
Type | Description |
---|---|
String | Anything inside double quotes. |
Number | Any number (no difference between ints and floats). |
Boolean | true and false . |
Null | JSON's empty value, denoted by null . |
Array | Like Python lists. |
Object | A collection of key-value pairs, like dictionaries. Keys must be strings, values can be anything (even other objects). |
See json-schema.org for more details.
import json
from pathlib import Path
f = Path('data') / 'family.json'
family_tree = json.loads(f.read_text())
family_tree
{'name': 'Grandma', 'age': 94, 'children': [{'name': 'Dad', 'age': 60, 'children': [{'name': 'Me', 'age': 24}, {'name': 'Brother', 'age': 22}]}, {'name': 'My Aunt', 'children': [{'name': 'Cousin 1', 'age': 34}, {'name': 'Cousin 2', 'age': 36, 'children': [{'name': 'Cousin 2 Jr.', 'age': 2}]}]}]}
family_tree['children'][0]['children'][0]['age']
24
Aside: eval
¶
eval
, which stands for "evaluate", is a function built into Python.It takes in a string containing a Python expression and evaluates it in the current context.
x = 3
eval('x * 10')
30
- It seems like
eval
can do the same thing thatjson.loads
does...
f.read_text()
'{\n "name": "Grandma",\n "age": 94,\n "children": [\n {\n "name": "Dad",\n "age": 60,\n "children": [{"name": "Me", "age": 24}, \n {"name": "Brother", "age": 22}]\n },\n {\n "name": "My Aunt",\n "children": [{"name": "Cousin 1", "age": 34}, \n {"name": "Cousin 2", "age": 36, "children": \n [{"name": "Cousin 2 Jr.", "age": 2}]\n }\n ]\n }\n ]\n}'
eval(f.read_text())
{'name': 'Grandma', 'age': 94, 'children': [{'name': 'Dad', 'age': 60, 'children': [{'name': 'Me', 'age': 24}, {'name': 'Brother', 'age': 22}]}, {'name': 'My Aunt', 'children': [{'name': 'Cousin 1', 'age': 34}, {'name': 'Cousin 2', 'age': 36, 'children': [{'name': 'Cousin 2 Jr.', 'age': 2}]}]}]}
- But you should almost never use
eval
. The next slide demonstrates why.
eval
gone wrong¶
Observe what happens when we use eval
on a string representation of a JSON object:
!cat data/evil_family.json
{ "name": "Grandma", "age": 94, "children": [ { "name": err(), "age": 60, "children": [{"name": "Me", "age": 24}, {"name": "Brother", "age": 22}] }, { "name": "My Aunt", "children": [{"name": "Cousin 1", "age": 34}, {"name": "Cousin 2", "age": 36, "children": [{"name": "Cousin 2 Jr.", "age": 2}] } ] } ] }
f_other = Path('data') / 'evil_family.json'
eval(f_other.read_text())
--------------------------------------------------------------------------- ValueError Traceback (most recent call last) Cell In[24], line 2 1 f_other = Path('data') / 'evil_family.json' ----> 2 eval(f_other.read_text()) File <string>:6 Cell In[1], line 5, in err() 4 def err(): ----> 5 raise ValueError('i just deleted all your files lol 😂') ValueError: i just deleted all your files lol 😂
Oh no! Since
evil_family.json
, which could have been downloaded from the internet, contained malicious code, we now lost all of our files.This happened because
eval
evaluates all parts of the input string as if it were Python code.You never need to do this – instead, use the
.json()
method of a response object, or use thejson
library.
Using the json
module¶
Let's process the same file using the json
module. Note:
json.load(f)
loads a JSON file from a file object.json.loads(f)
loads a JSON file from a string.
f_other = Path('data') / 'evil_family.json'
s = f_other.read_text()
s
'{\n "name": "Grandma",\n "age": 94,\n "children": [\n {\n "name": err(),\n "age": 60,\n "children": [{"name": "Me", "age": 24}, \n {"name": "Brother", "age": 22}]\n },\n {\n "name": "My Aunt",\n "children": [{"name": "Cousin 1", "age": 34}, \n {"name": "Cousin 2", "age": 36, "children": \n [{"name": "Cousin 2 Jr.", "age": 2}]\n }\n ]\n }\n ]\n}'
print(s)
{ "name": "Grandma", "age": 94, "children": [ { "name": err(), "age": 60, "children": [{"name": "Me", "age": 24}, {"name": "Brother", "age": 22}] }, { "name": "My Aunt", "children": [{"name": "Cousin 1", "age": 34}, {"name": "Cousin 2", "age": 36, "children": [{"name": "Cousin 2 Jr.", "age": 2}] } ] } ] }
json.loads(s)
--------------------------------------------------------------------------- JSONDecodeError Traceback (most recent call last) Cell In[27], line 1 ----> 1 json.loads(s) File ~/miniforge3/envs/dsc80/lib/python3.8/json/__init__.py:357, in loads(s, cls, object_hook, parse_float, parse_int, parse_constant, object_pairs_hook, **kw) 352 del kw['encoding'] 354 if (cls is None and object_hook is None and 355 parse_int is None and parse_float is None and 356 parse_constant is None and object_pairs_hook is None and not kw): --> 357 return _default_decoder.decode(s) 358 if cls is None: 359 cls = JSONDecoder File ~/miniforge3/envs/dsc80/lib/python3.8/json/decoder.py:337, in JSONDecoder.decode(self, s, _w) 332 def decode(self, s, _w=WHITESPACE.match): 333 """Return the Python representation of ``s`` (a ``str`` instance 334 containing a JSON document). 335 336 """ --> 337 obj, end = self.raw_decode(s, idx=_w(s, 0).end()) 338 end = _w(s, end).end() 339 if end != len(s): File ~/miniforge3/envs/dsc80/lib/python3.8/json/decoder.py:355, in JSONDecoder.raw_decode(self, s, idx) 353 obj, end = self.scan_once(s, idx) 354 except StopIteration as err: --> 355 raise JSONDecodeError("Expecting value", s, err.value) from None 356 return obj, end JSONDecodeError: Expecting value: line 6 column 17 (char 84)
Since
util.err()
is not a string in JSON (there are no quotes around it),json.loads
is not able to parse it as a JSON object.This "safety check" is intentional.
Handling unfamiliar data¶
Never trust data from an unfamiliar site.
Never use
eval
on "raw" data that you didn't create!The JSON data format needs to be parsed, not evaluated as a dictionary.
- It was designed with safety in mind!
Aside: pd.read_json
¶
pandas
also has a built-in read_json
function.
pd.read_json(f)
name | age | children | |
---|---|---|---|
0 | Grandma | 94 | {'name': 'Dad', 'age': 60, 'children': [{'name... |
1 | Grandma | 94 | {'name': 'My Aunt', 'children': [{'name': 'Cou... |
It only makes sense to use it, though, when you have a JSON file that has some sort of tabular structure. Our family tree example does not.
APIs and scraping¶
Programmatic requests¶
We learned how to use the Python
requests
package to exchange data via HTTP.GET
requests are used to request data from a server.POST
requests are used to send data to a server.
There are two ways of collecting data through a request:
- By using a published API (application programming interface).
- By scraping a webpage to collect its HTML source code.
APIs¶
An application programming interface (API) is a service that makes data directly available to the user in a convenient fashion.
Advantages:
The data are usually clean, up-to-date, and ready to use.
The presence of a API signals that the data provider is okay with you using their data.
The data provider can plan and regulate data usage.
- Some APIs require you to create an API "key", which is like an account for using the API.
- APIs can also give you access to data that isn't publicly available on a webpage.
Big disadvantage: APIs don't always exist for the data you want!
API terminology¶
A URL, or uniform resource locator, describes the location of a website or resource.
An API endpoint is a URL of the data source that the user wants to make requests to.
For example, on the Reddit API:
- the
/comments
endpoint retrieves information about comments. - the
/hot
endpoint retrieves data about posts labeled "hot" right now.
- To access these endpoints, you add the endpoint name to the base URL of the API.
- the
API requests¶
- API requests are just
GET
/POST
requests to a specially maintained URL. - Let's test out the Pokémon API.
First, let's make a GET
request for 'squirtle'
. To do this, we need to make a request to the correct URL.
def create_url(pokemon):
return f'https://pokeapi.co/api/v2/pokemon/{pokemon}'
create_url('squirtle')
'https://pokeapi.co/api/v2/pokemon/squirtle'
r = requests.get(create_url('squirtle'))
r
<Response [200]>
Remember, the 200 status code is good! Let's take a look at the content:
r.content[:1000]
b'{"abilities":[{"ability":{"name":"torrent","url":"https://pokeapi.co/api/v2/ability/67/"},"is_hidden":false,"slot":1},{"ability":{"name":"rain-dish","url":"https://pokeapi.co/api/v2/ability/44/"},"is_hidden":true,"slot":3}],"base_experience":63,"forms":[{"name":"squirtle","url":"https://pokeapi.co/api/v2/pokemon-form/7/"}],"game_indices":[{"game_index":177,"version":{"name":"red","url":"https://pokeapi.co/api/v2/version/1/"}},{"game_index":177,"version":{"name":"blue","url":"https://pokeapi.co/api/v2/version/2/"}},{"game_index":177,"version":{"name":"yellow","url":"https://pokeapi.co/api/v2/version/3/"}},{"game_index":7,"version":{"name":"gold","url":"https://pokeapi.co/api/v2/version/4/"}},{"game_index":7,"version":{"name":"silver","url":"https://pokeapi.co/api/v2/version/5/"}},{"game_index":7,"version":{"name":"crystal","url":"https://pokeapi.co/api/v2/version/6/"}},{"game_index":7,"version":{"name":"ruby","url":"https://pokeapi.co/api/v2/version/7/"}},{"game_index":7,"version":{"nam'
Looks like JSON. We can extract the JSON from this request with the json
method (or by passing r.text
to json.loads
).
rr = r.json()
# rr # Hidden in the HTML version of the lecture notebook because it's way too long.
rr.keys()
dict_keys(['abilities', 'base_experience', 'forms', 'game_indices', 'height', 'held_items', 'id', 'is_default', 'location_area_encounters', 'moves', 'name', 'order', 'past_abilities', 'past_types', 'species', 'sprites', 'stats', 'types', 'weight'])
rr['weight']
90
rr['stats']
[{'base_stat': 44, 'effort': 0, 'stat': {'name': 'hp', 'url': 'https://pokeapi.co/api/v2/stat/1/'}}, {'base_stat': 48, 'effort': 0, 'stat': {'name': 'attack', 'url': 'https://pokeapi.co/api/v2/stat/2/'}}, {'base_stat': 65, 'effort': 1, 'stat': {'name': 'defense', 'url': 'https://pokeapi.co/api/v2/stat/3/'}}, {'base_stat': 50, 'effort': 0, 'stat': {'name': 'special-attack', 'url': 'https://pokeapi.co/api/v2/stat/4/'}}, {'base_stat': 64, 'effort': 0, 'stat': {'name': 'special-defense', 'url': 'https://pokeapi.co/api/v2/stat/5/'}}, {'base_stat': 43, 'effort': 0, 'stat': {'name': 'speed', 'url': 'https://pokeapi.co/api/v2/stat/6/'}}]
rr['moves'][26]['move']
{'name': 'double-team', 'url': 'https://pokeapi.co/api/v2/move/104/'}
requests.get('https://pokeapi.co/api/v2/move/104/').json()
Let's try a GET
request for 'billy'
.
r = requests.get(create_url('billy'))
r
<Response [404]>
We receive a 404 error, since there is no Pokemon named 'billy'
!
Scraping¶
Scraping is the act of programmatically "browsing" the web, downloading the source code (HTML) of pages that you're interested in extracting data from.
Big advantage: You can always do it! For example, Google scrapes webpages in order to make them searchable.
Disadvantages:
It is often difficult to parse and clean scraped data.
- Source code often includes a lot of content unrelated to the data you're trying to find (e.g. formatting, advertisements, other text).
Websites can change often, so scraping code can get outdated quickly.
Websites may not want you to scrape their data!
In general, we prefer APIs, but scraping is a useful skill to learn.
Example: Scraping the HDSI faculty page¶
To fully understand how to scrape, we need to understand how HTML documents are structured and how to extract information out of them.
But as a preview of what's to come next week, let's start by making a request to the HDSI Faculty page, https://datascience.ucsd.edu/faculty.
fac_response = requests.get('https://datascience.ucsd.edu/faculty/')
fac_response
<Response [200]>
The response is a long HTML document.
len(fac_response.text)
277027
print(fac_response.text[:3000])
<!DOCTYPE html> <html lang="en-US"> <head> <meta charset="UTF-8"> <meta name="viewport" content="width=device-width, initial-scale=1.0" /> <meta http-equiv="X-UA-Compatible" content="IE=edge"> <link rel="profile" href="https://gmpg.org/xfn/11" /> <title>Faculty – Halıcıoğlu Data Science Institute – UC San Diego</title> <script> /* You can add more configuration options to webfontloader by previously defining the WebFontConfig with your options */ if ( typeof WebFontConfig === "undefined" ) { WebFontConfig = new Object(); } WebFontConfig['google'] = {families: ['Jost:400,700', 'Roboto:400,500']}; (function() { var wf = document.createElement( 'script' ); wf.src = 'https://ajax.googleapis.com/ajax/libs/webfont/1.5.3/webfont.js'; wf.type = 'text/javascript'; wf.async = 'true'; var s = document.getElementsByTagName( 'script' )[0]; s.parentNode.insertBefore( wf, s ); })(); </script> <meta name='robots' content='max-image-preview:large' /> <link rel='dns-prefetch' href='//kit.fontawesome.com' /> <link rel='dns-prefetch' href='//platform-api.sharethis.com' /> <link rel='dns-prefetch' href='//fonts.googleapis.com' /> <link rel='dns-prefetch' href='//use.fontawesome.com' /> <link rel="alternate" type="application/rss+xml" title="Halıcıoğlu Data Science Institute - UC San Diego » Feed" href="https://datascience.ucsd.edu/feed/" /> <link rel="alternate" type="text/calendar" title="Halıcıoğlu Data Science Institute - UC San Diego » iCal Feed" href="https://datascience.ucsd.edu/events/?ical=1" /> <!-- This site uses the Google Analytics by MonsterInsights plugin v8.23.1 - Using Analytics tracking - https://www.monsterinsights.com/ --> <script src="//www.googletagmanager.com/gtag/js?id=G-ZWZDLH05C7" data-cfasync="false" data-wpfc-render="false" type="text/javascript" async></script> <script data-cfasync="false" data-wpfc-render="false" type="text/javascript"> var mi_version = '8.23.1'; var mi_track_user = true; var mi_no_track_reason = ''; var disableStrs = [ 'ga-disable-G-ZWZDLH05C7', ]; /* Function to detect opted out users */ function __gtagTrackerIsOptedOut() { for (var index = 0; index < disableStrs.length; index++) { if (document.cookie.indexOf(disableStrs[index] + '=true') > -1) { return true; } } return false; } /* Disable tracking if the opt-out cookie exists. */ if (__gtagTrackerIsOptedOut()) { for (var index = 0; index < disableStrs.length; in
To parse HTML, we'll use the BeautifulSoup library.
from bs4 import BeautifulSoup
soup = BeautifulSoup(fac_response.text)
Now, soup
is a representation of the faculty page's HTML code that Python knows how to extract information from.
# Magic that we'll learn how to create together next Tuesday.
divs = soup.find_all('div', class_='vc_grid-item')
names = [div.find('h4').text for div in divs]
titles = [div.find(class_='pendari_people_title').text for div in divs]
faculty = pd.DataFrame({
'name': names,
'title': titles,
})
faculty.head()
name | title | |
---|---|---|
0 | Ilkay Altintas | SDSC Chief Data Science Officer & HDSI Foundin... |
1 | Tiffany Amariuta | Assistant Professor |
2 | Mikio Aoi | Assistant Professor |
3 | Ery Arias-Castro | Professor |
4 | Vineet Bafna | Professor |
Now we have a DataFrame!
faculty[faculty['title'].str.contains('Lecturer') | faculty['title'].str.contains('Teaching')]
name | title | |
---|---|---|
12 | Justin Eldridge | Assistant Teaching Professor |
13 | Shannon Ellis | Associate Teaching Professor |
27 | Marina Langlois | Lecturer |
... | ... | ... |
39 | Suraj Rampure | Lecturer |
47 | Jack Silberman | Lecturer |
51 | Janine Tiefenbruck | Lecturer |
9 rows × 2 columns
What if we want to get faculty members' pictures?
from IPython.display import Image, display
def show_picture(name):
idx = faculty[faculty['name'].str.lower().str.contains(name.lower())].index[0]
display(Image(divs[idx].find('img')['src'], width=200, height=200))
show_picture('justin')
Midterm review¶
You'll need to look at the podcast for this part.
The plan¶
We'll work with a single dataset and try and revisit some of the most commonly requested ideas using it.
The data is (mostly) real; each row is a student from the Spring 2023 offering of DSC 10.
students_path = Path('data') / 'dsc10-sp23-roster.csv'
students = pd.read_csv(students_path)
students.head()
Section | IG Followers | Unread Emails | College | Major | Class Standing | |
---|---|---|---|---|---|---|
0 | A | 659 | 5 | Revelle | CM26 | Senior |
1 | B | 814 | 11320 | Warren | MA33 | Senior |
2 | B | 616 | 7340 | Revelle | MA30 | Junior |
3 | A | 278 | 0 | Seventh | HI25 | Sophomore |
4 | A | 182 | 4664 | ERC | EN25 | Senior |
How many students are there in each college?
students.groupby('College').size()
College ERC 24 Marshall 31 Muir 20 Revelle 31 Seventh 35 Sixth 49 Warren 38 dtype: int64
students.groupby('College').count()
Section | IG Followers | Unread Emails | Major | Class Standing | |
---|---|---|---|---|---|
College | |||||
ERC | 24 | 24 | 24 | 24 | 24 |
Marshall | 31 | 31 | 31 | 31 | 31 |
Muir | 20 | 20 | 20 | 20 | 20 |
Revelle | 31 | 31 | 31 | 31 | 31 |
Seventh | 35 | 35 | 35 | 35 | 35 |
Sixth | 49 | 49 | 49 | 49 | 49 |
Warren | 38 | 38 | 38 | 38 | 38 |
students['College'].value_counts()
Sixth 49 Warren 38 Seventh 35 Revelle 31 Marshall 31 ERC 24 Muir 20 Name: College, dtype: int64
How many students in each college have over 1000 followers?
students
Section | IG Followers | Unread Emails | College | Major | Class Standing | |
---|---|---|---|---|---|---|
0 | A | 659 | 5 | Revelle | CM26 | Senior |
1 | B | 814 | 11320 | Warren | MA33 | Senior |
2 | B | 616 | 7340 | Revelle | MA30 | Junior |
... | ... | ... | ... | ... | ... | ... |
225 | B | 251 | 4729 | Sixth | PB25 | Junior |
226 | B | 490 | 0 | ERC | CG35 | Junior |
227 | A | 844 | 0 | Revelle | MC25 | Sophomore |
228 rows × 6 columns
(
students[students['IG Followers'] >= 1000]
['College']
.value_counts()
)
Marshall 6 Sixth 6 Revelle 5 Warren 3 ERC 3 Muir 1 Name: College, dtype: int64
(
students
.groupby('College')
['IG Followers']
.agg(lambda s: (s >= 1000).sum())
.sort_values(ascending=False)
)
College Marshall 6 Sixth 6 Revelle 5 ERC 3 Warren 3 Muir 1 Seventh 0 Name: IG Followers, dtype: int64
Which colleges have at least 25 students that have over 20 unread emails?
def college_filter(df):
return (df['Unread Emails'] > 20).sum() >= 25
(
students
.groupby('College')
.filter(college_filter)
['College']
.unique()
)
array(['Sixth'], dtype=object)
college_filter(students[students['College'] == 'Sixth'])
True
(
students
.groupby('Section')
.mean()
['IG Followers']
)
Section A 451.18 B 491.57 Name: IG Followers, dtype: float64
(
students
.groupby('Section')
['IG Followers']
.mean()
)
Section A 451.18 B 491.57 Name: IG Followers, dtype: float64
students
Section | IG Followers | Unread Emails | College | Major | Class Standing | |
---|---|---|---|---|---|---|
0 | A | 659 | 5 | Revelle | CM26 | Senior |
1 | B | 814 | 11320 | Warren | MA33 | Senior |
2 | B | 616 | 7340 | Revelle | MA30 | Junior |
... | ... | ... | ... | ... | ... | ... |
225 | B | 251 | 4729 | Sixth | PB25 | Junior |
226 | B | 490 | 0 | ERC | CG35 | Junior |
227 | A | 844 | 0 | Revelle | MC25 | Sophomore |
228 rows × 6 columns
def is_cool(row):
if row['Section'] == 'A' and row['College'] == 'Warren':
return 'yes'
elif row['Section'] == 'B' and row['Class Standing'] != 'Junior':
return 'yes'
elif np.random.random() < 0.5:
return 'idk'
else:
return 'no'
students.apply(
is_cool,
axis=1
)
0 no 1 yes 2 idk ... 225 idk 226 idk 227 no Length: 228, dtype: object
%%timeit
students['IG Followers'] + students['Unread Emails']
25.8 µs ± 166 ns per loop (mean ± std. dev. of 7 runs, 10,000 loops each)
Hypothesis and permutation testing¶
students
Section | IG Followers | Unread Emails | College | Major | Class Standing | |
---|---|---|---|---|---|---|
0 | A | 659 | 5 | Revelle | CM26 | Senior |
1 | B | 814 | 11320 | Warren | MA33 | Senior |
2 | B | 616 | 7340 | Revelle | MA30 | Junior |
... | ... | ... | ... | ... | ... | ... |
225 | B | 251 | 4729 | Sixth | PB25 | Junior |
226 | B | 490 | 0 | ERC | CG35 | Junior |
227 | A | 844 | 0 | Revelle | MC25 | Sophomore |
228 rows × 6 columns
How does the distribution of IG followers for section A compare to section B?
students.groupby('Section')['IG Followers'].mean()
Section A 451.18 B 491.57 Name: IG Followers, dtype: float64
# create_kde_plotly(
# students,
# 'Section',
# 'A',
# 'B',
# 'IG Followers'
# )
Permutation tests help us decide whether the differences between two samples are significant.
students.groupby('Section')['IG Followers'].describe()
count | mean | std | min | 25% | 50% | 75% | max | |
---|---|---|---|---|---|---|---|---|
Section | ||||||||
A | 115.0 | 451.18 | 408.88 | 0.0 | 102.5 | 326.0 | 752.0 | 1772.0 |
B | 113.0 | 491.57 | 797.11 | 0.0 | 59.0 | 251.0 | 620.0 | 6160.0 |
ig = students[['Section', 'IG Followers']]
ig
Section | IG Followers | |
---|---|---|
0 | A | 659 |
1 | B | 814 |
2 | B | 616 |
... | ... | ... |
225 | B | 251 |
226 | B | 490 |
227 | A | 844 |
228 rows × 2 columns
Null: The distribution of IG followers for students in section A is the same as the distribution of IG followers for students in section B.
Alternative: Students in section B have more IG followers on average (mean) than students in section B.
students.groupby('Section')['IG Followers'].describe()
count | mean | std | min | 25% | 50% | 75% | max | |
---|---|---|---|---|---|---|---|---|
Section | ||||||||
A | 115.0 | 451.18 | 408.88 | 0.0 | 102.5 | 326.0 | 752.0 | 1772.0 |
B | 113.0 | 491.57 | 797.11 | 0.0 | 59.0 | 251.0 | 620.0 | 6160.0 |
test statistic:
difference in group means
ig
Section | IG Followers | |
---|---|---|
0 | A | 659 |
1 | B | 814 |
2 | B | 616 |
... | ... | ... |
225 | B | 251 |
226 | B | 490 |
227 | A | 844 |
228 rows × 2 columns
ig.groupby('Section')['IG Followers'].mean()
Section A 451.18 B 491.57 Name: IG Followers, dtype: float64
ig.groupby('Section')['IG Followers'].mean().diff()
Section A NaN B 40.38 Name: IG Followers, dtype: float64
obs = ig.groupby('Section')['IG Followers'].mean().diff().iloc[-1]
obs
40.38376298576378
stats = []
for _ in range(10000):
ig['Section'] = np.random.permutation(ig['Section'])
stat = ig.groupby('Section')['IG Followers'].mean().diff().iloc[-1]
stats.append(stat)
pd.Series(stats).plot(kind='hist')
x-axis is group B - group A.
obs
40.38376298576378
(
students
.pivot_table(
index='College',
columns='Section',
values='IG Followers',
aggfunc='count'
)
.pipe(lambda df: df / df.sum())
.diff(axis=1)
.abs()
.iloc[:, 1]
.sum() / 2
)
0.22146979607541362
students[['Section', 'College']]
Section | College | |
---|---|---|
0 | A | Revelle |
1 | B | Warren |
2 | B | Revelle |
... | ... | ... |
225 | B | Sixth |
226 | B | ERC |
227 | A | Revelle |
228 rows × 2 columns